Crafting Ethical AI Products and Services – a UX Guide
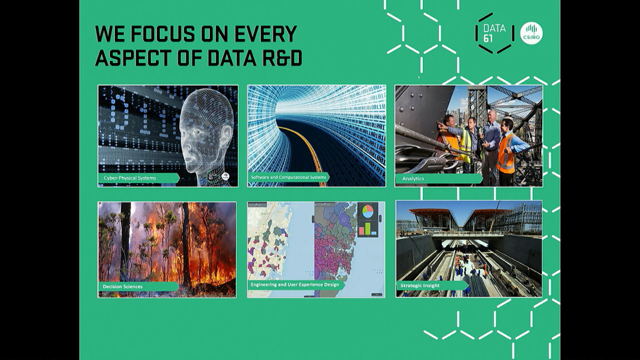
(upbeat music) - So I'm Hilary Cinis.
I head up the Design and User Experience and Design group at Data 61 which is now a business unit of CSIRO. Previously we were NICTA and the digital productivity flagship at CSIRO. So I'm going to talk about how to craft digital products and services that have ethical outcomes for society. And I don't have a cookie-cutter or a solution for you. It's actually proposed methods and some frameworks. That way.
Inability to drive.
Okay, so who's CSIRO and who are Data 61? I'm assuming everyone knows who CSIRO is.
It's the national science and technology research organisation.
It's been around for about 100 years.
Agriculture, health, biosecurity, oceans and atmosphere, and recently technology innovation.
So it's an emerging sort of capability within our organisation.
Data 61, we have our own special brand to help kind of reestablish that foothold in industry as innovation technology hothouse.
About 1,000 employees, lots of students, lots of government partners, lots of corporate partners, university, over a 190 projects, and an ongoing list of patents.
I'm sure this is out of date already.
And what we do there is we kind of focus on every aspect of the data journey.
That's from collection through to measuring, and synthesising, and creating inferences, and making decision support, right out to outputs for users and actually out into industry as well. And we focus on every aspect of R&D.
So we have cyber physical systems, which is autonomous and robots working in manufacture and also in industry as well, mining, et cetera.
Software and computational systems.
Of course, we're doing blockchaining like everybody else, along with AI and machine learning.
Analytics are getting information out of the data, large data, small data, private data, open data. Decision sciences, forecasting, predictions. Engineering and User Experience Design which is the team that we are in and also strategic insights.
So we have a large group of people who do forecasting around trends, mega trends, future states, future industries.
We kind of bring all that together in different ways. The User Experience and Design team as I mentioned sits within the engineering group, and our primary focus is to ensure meaningful interactions between the people who are looking for information and the people who are making information.
So there are a whole bunch of people who are collecting data and producing technologies and softwares and solutions and there are a whole bunch of people who need assistance to make decisions with using their technology.
And so our job is to help make sure that the APIs are meaningful, that software works, and that there's like an ecosystem approach to this.
So we're not really here to build products and suites of products, we're here to build an ecosystem upon which other people can build their products and their technologies as well.
But we do push out early prototypes, to elevate that work ongoing.
So there's two parts to this talk.
The first one is positioning and the second one is a whole bunch of methods proposed that you may take or leave in practise as UX designers. And I just want to say I'm not a philosopher or ethicist by training.
I'm an arm chair mug who just likes this stuff and tries to read about it all as much as I can. So there's basically two kinds of philosophical schools. There's utilitarianism which is kind of like for the greater good where big decisions are made because it's like it affects a large number of people. And there's deontological which is a sense of duty. So you know poor old John McClane was stuck in Nakatomi Tower.
He really didn't like what he had to do, but he had to save all those people.
So he had a sense of duty.
So these are kind of philosophical schools. They're not mutually exclusive.
In fact, they work together all the time, and that's the whole, you know.
There's talk of ethics in technology, very, very easily flips over into philosophy before you know it.
Any time you try to make decisions about values, about who's being harmed, who's being left behind, what's the purpose of the work that we are doing it really, really easily steps backwards into what are the values, what are our beliefs, what is it we're trying to achieve, what are our intentions. So before we go any further, and I probably don't need to remind anybody about this, but I'm going to say it.
AI and machine learning does not have its own agency. It's everything that's created, all technology is created by humans.
So if you're talking to people, remind them of that. If people start talking about the machines, or the AI, or whatever it is and getting freaked out about it.
Humans are making that stuff.
We are making that stuff.
We have control over it, and I don't think the machines gonna take over the world.
I think it will just be ubiquitous and pervasive like cars are and electricity is.
If anyone doesn't know the reference to this, (laughs) this big rock monster comes alive at the will of these creatures, and then try to kill Commander Taggart in Galaxy Quest. It's not actually a live thing.
Okay, so why do we have an ethical practise for technology? And what informs an ethical practise? And how achievable is it? It's messy and it's hard, and I reckon for the first time UX people have got this enormous opportunity to really step forward and say you know what? We are uniquely well-positioned for this work because our entire reason of being is to think about somebody else using the products, someone else using the services, how easy it is for them, how painful it is for them, and we can just extend our natural skill sets into asking these questions early and constantly throughout our work.
And if technologists really and big companies really do care about reduction of harm, they've got a stack of people in house who are already prepared to do that work, so take advantage of it, make some noise, and hopefully they'll listen.
So this is from two quotes about the timing of this. The IEEE and AI Now.
If you don't know who they are, I strongly suggest you look them up and start reading about their frameworks.
They've proposed a whole bunch of really good pieces of work and some good ideas for frameworks to go forward for government, for industry, oops. For government, for industry, and practitioners as well. So, IEEE talk about diverse teams, multidisciplinary teams tackling this issue from school right through to deep, you know, people who have been producing products for long time, so new products, established products, getting in early, university, how to train current practitioners, and how to prepare for the future.
It's very holistic.
They've proposed that the research and design methods must be underpinned by ethical and legal norms as well as methods.
So it's normalising this mindset that's really important, and then that moves forward into regulatry as well because we know that you can't like appeal to a psychopath or a sociopath.
There are gonna be people who will do the wrong thing all the time, and that's why there needs to be regulatry frameworks as well as good intention and mindfulness by the people who are doing the work. So they're proposing a value-based design methodology and what that looks like we'll talk about a bit more in a minute.
Putting a human advancement at the core of things. So it's aspirational, this is highly aspirational stuff and that's a good thing, right? You can't just sort of write it off.
It gets depressing at times.
I sit there going what's the point, I'm watching the news and this Trump being a dick and everybody else carrying on.
It's like this is irrelevant, but it's like no, no, no. Having an aspiration is part of human nature and it's a good thing.
We want to create sustainable systems, and they should be scrutinised and then we can defend them. And it's okay to make mistakes, right? As long as it's upfront, and there's an order trail, and everyone knows why the decisions were being made. That's all that's really being asked for here, consideration clearly stated.
And human rights form it as well.
So there's actually some existing, really well structured, well thought through positions that all of this stuff can draw from.
It's not brand new.
There are heaps of people working on this right now. And the AI Now report's great 'cause too often user consent, privacy, and transparency are overlooked in favour of frictionless functionality.
Now this report came out way before the Facebook debacle, so it's really timely.
And this topic about profit-driven business models is really key, so we cannot sit around railing at what's going on, but we've all cried ourselves to sleep as some point, right? Because the business has asked us to do things that we didn't agree with or we didn't win that fight or we had to make too many compromises and we knew that the outcome wasn't going to be good. So this clearly states that it comes from the top down. And there've been discussions about changing how boards of directors and how business KPI's need to adjust as well in order to accommodate social good and not just are relentless pursuit of money. So there's not going to be a hard and fast answer to this question.
So we look for an ethical practise rather than the definition of an ethical product. Our products are always evolving, they're always iterating, there's always new opportunities, and so every single time we start to do something, we need to ask why and for whom.
And on the topic of data, which all of this sits on top of, there's the trust versus utility trade off. So private data, highly personal data is extremely valuable. And the more open the data is, its utility becomes less. You can do good things with it, but it's less. Somehow Facebook has managed to make public data out of private data which has increased its utility, so that's what's happened there.
So we need to capture upfront and measure the ethical implications.
So trade-offs is really important here.
And there will always be compromises, and there will always be trade-offs.
How useful is this information? Do we get more private data? How do we protect that so we can make better decisions out of that data? Do we use more public data and have it less useful? Other people can then build on top of it.
What are the trade-offs there? And there's also some topics here around you know, like judging, like sentencing algorithms which I'm sure Sara talked about yesterday. Perhaps it's okay for some small cohorts of offenders under a certain kind of qualification to be have an automated sentencing algorithm run over them. I don't know.
But and the rest of them need to have human in the loop or entirely a human sort of judged outcome. But if it can be understood why 20% are being put through an automatic position and they have a right of reply, then that's okay but the trade-off is like there's efficiency versus harm.
And that's the trade-off at all times.
There are computer scientists and machine learning practitioners who are trying to encode fairness into algorithms. And if you're interested in that, look up Bob Williamson from ANU.
He talked about it earlier this year at the FAT Conference, the Fairness, Accountability, and Transparency Conference.
So the computer scientists are working at encoding fairness and ethics into their logic, but we're not quite there yet.
So there's a lot of, this is why we are an important part of this, in investigating it, getting to the bottom of the intentions, getting to the bottom of the trade-off.
It's literally asking that, you know, the founder of that start-up, or the CEO, or that department head of an agency, what number are you going to give to the people who get left behind? Is it okay that 20% of people in low socioeconomic areas are harmed by this outcome if 80% have an improvement. And if they're uncomfortable with that, then that's a really good way of finding out if it's actually a good or bad an idea to go ahead with the work.
The use of affordances and cultural conventions and visual feedback and signifiers. This talks in the solutions part of it, when people are interacting with the technology. So they understand how the systems are working. That they can interact with these systems.
The machines are making decisions on their behalf. If I'm a data analyst, and I'm using a piece of software to analyse large groups of people in a bank to make some decisions about I don't know credit rating and therefore selling them insurance.
Is it talking to me in a language I understand or is it acting like a complete foreign entity? Which is often a problem we've come across when we've been proposing solutions for data scientists that we work with.
They understand that the math is really solid and they understand the machines are doing really good things, but it's not giving it back to them in a language they identify in that they understand. And when we talk about the black box of algorithms, this is what this is.
The black box of machine learning.
People don't understand how it's working because they can't sort of see how to tweak the knobs and get in there and change the values in a way that makes sense to them.
Really important that the social expectations are included. The broader the user base, obviously the more variety of individuals that are going to be interacting with the software. And again I missed Sara's talk, so I'm really sorry if I'm referencing her kind of second hand. But in her book, she talks about edge cases, and how edge cases actually is How edge cases are not a great thing and not a great phrase. Everyone's an edge case at some point.
So the bigger your user base, the more consideration this takes.
And how do we deliver outcomes that foster and reward trust? I think Nick's going to talk a lot about trust after this, so I'm not going to cover that too much.
So this is a collective activity.
It's not just us, it's not just the software designers, software developers.
In a lot of the literature at the moment, I'm seeing the word designer and I'm assuming that that's product teams, not actual designers themselves being sort of held up as the people to sort of resolve this problem.
But an ethical approach to technology is an entirely collective activity, and it starts with the law.
We actually have laws about this.
And if you're, and you've got legal people in your own departments, you can speak to them and get them on board.
How we used to get the developers on board with us to help us reach our objectives, it's time to start wooing the legal department as well 'cause they're the ones that are going to feel it, right? When the law comes knocking if somethings gone wrong. And if you're lucky enough to have a cooperate ethics group which at CSIRO we do, they're obviously involved as well.
And they answer the question, "Can we?" Can we do this? And if it comes back that legally you can't do it, then you shouldn't do it.
You should not be profiling social security recipients for drug use using machines.
That is actually illegal.
And unethical.
Should we? So the business, this is where the business asks the questions.
Should we be doing this work? Is it going to give us a commercial return? Is it worth the investment of effort? If we have to dig into this new innovation, and we have to do all of this legal and ethical activities, is it really worth our time doing it and are we going to get a good advantage out of it? And if they say yes, that's great.
And then business can go and find some clients, or find some new markets, whatever they're doing. By the time it gets down to us, we're asking who and why. That is our job, and the devs our asking how. How are they going to build it? So underneath all of this is the data.
Legally speaking there are standards and governances around data collection and data use.
The executive looking at the utility which we spoke about before.
How useful is it? How much decisions are we going to get out of it? How much money are we going to make out of it? What's the value of the work? And we're looking at the data itself.
How it's being measured, how complete it is, and what decisions that can be made out of that data. And then who is going to benefit from that as well. So we're covering that kind of deontological aspect of it which is the duty.
And the software devs are looking at the utilitarian aspect of it which is the encoding of it, giving it a number because that's how the software's working currently.
And that's a big feedback loop as well.
If it comes down to, if one of these decisions are unclear and obviously if it comes to us and we're like what problem is it solving and for whom and if that can't be answered, well then clearly there's no business case. And if the devs are kind of going we literally cannot encode this, this is just too messy, there needs to be less programming and more skills training perhaps in the users that are going to be using it. Then that's an outcome as well.
So you know, not everything, not every technology is a fully digital solution.
There might be completely other aspects to the business. Similarly to the design systems, it's like a whole ecosystem of interactions around it as well that have to be recreated. So where does this leave us as designers? We are being held up apparently as either responsible or as the champions, and a note, Ari Roth, South by Southwest, says apparently we're blindly just going ahead and doing whatever we want.
It's a little 'd' not a big 'd', so I am assuming that means product teams, but you know it kind of rankled me when I read this to be honest.
I just immediately took offence.
(audience laughs) Yes, that happened to me.
And Evan Selinger in a recent article talked about design issues are wearing away the public's trust. So apparently again, we're doing a bad job, but I'm assuming that's meant to be technology teams not designers per se. So the people that are making the technology, actually making the technology, none of these people are talking about the business decisions that drive technology production.
We're being looked at.
We're under scrutiny at the moment.
And I reckon that's great 'cause we can go, you know, we need help.
The business, you need to help me do my job properly. I can do this job, I can do this job well, help me out with it.
So it's kind of two phases for UX people to get involved, and obviously there's people who perform uses and design as a practise and there are people who perform uses and design as a shared mindset.
So the project teams, project owners, project managers, developers, all of these people either have it as a mindset or they actually have it as an actual practise. So I am throwing out a lot wider than designers but really uses it as a design here.
'Cause there's what happens at the discovery phase and there's what happens in the user communication phase which is the solution end of things.
And the good news is it's just our typical skill set with just a bit of an extra lens on top.
It's actually not that big a leap for us to do this work. We're always asking who and why before how and what. We have this kind of, we don't have assigners because the work that we do is so varied and we don't do suites of products like I mentioned before, but we have kind of like reoccurring user types that we refer to.
So the most powerful person in this relationship between technology and ethics is the one with the money, and that would be the enabler, or the project sponsor, and that's usually like a department head, a CEO, a programme manager, a founder in a start-up, someone who's got the vision, and the mission, and the drive, and the money.
They've got the most power in this relationship, but they're the most far away from the recipient which is the like the customer or the citizen. That poor person at the end of the process who's trying to get through the day, make decisions, be sold something, whatever.
And I've deliberately inverted this so that the person with the least amount of power in the relationship is now at the top as the focus, and the one with the most is at the bottom. We have technical experts, software engineers, data scientists who are building technology. We are sometimes tech experts as well because we are helping to build this tech.
Some kind of an ethical data service has been set up. It's pulling in private data from the users and products being built, and sometimes what we call an engaged professional is delivering a service to somebody.
So if you think about this as being the enabler, this is someone at department of human services, and they've asked some software people to build some sort of system.
Now that might be a Data 61 engineer or it could be someone within DHS.
We've got a single parent on welfare at the top here. Their data's being used to populate this information. They go to, I don't know why I'm standing like this, sorry. They hop along to, you know, services in New South Wales to get some information about their account and that's where the engaged professional is. The data analyst, or a consultant, or service provider is giving you information about your life through this system.
So if they're making decisions on your behalf, and you don't understand those decisions, you don't like those decisions, you need to be able to, they need to explain it. They probably can't explain it.
In fact, probably only this person can explain it, and this needs to stop.
This is a lack of control and a lack of respect. So I think it's up to us with our natural skill-sets which we do when we do service design and all the things which we do to explain the relationships between the users and where the power can be abused and where the benefits are.
And understanding the data and contextual use for each type. You know the technology, tech expert might actually see private data but because they're in a secure enclave or the kind of technologies that they're using to reach it protects this person from being identified. It's either by law or through technology.
The engaged professional, they're not getting all that information.
They're getting some kind of aggregated inference that comes out of it.
And then the recipient gets the knowledge.
So what is the trade-off here? You know, how much how private information should the engaged professional have compared to the citizen and how comfortable would they be? And of course, there's cultural ethics as well. It's a really important part of this.
English as a second language, disadvantaged groups, they all need to be considered.
They're no longer edge cases, and they're no longer not the primary customer. So the other end of things, we've got the user communications.
So as I mentioned before, there's data measurement, there's data completeness, and there's inferencing. If the source of the data is wrong or poorly measured or poorly collected or historically biassed, don't need to talk much about that, I'm sure that's been talked about a lot this week, there's going to be issues.
And if there's any disagreement with that, the credibility goes out the window.
If the precision is okay but the completeness is dodgy, if it's full of gaps and nothing's been made to kind of address those gaps disaggregation instead of you know nominating that there are gaps in the data, there's going to be issues with the outcomes as well. And if the inferencing is bad, if one or two are okay, but the decisions that are coming out of it are not well considered for the users, then you're going to have disagreement too. So I had this exact problem.
I did a job for the environmental protection agency. The data was being collected nicely at the source with census.
It was incomplete because it was kind of trying to aggregate across space and time.
It was spacial-temporal prediction platform. So there were big gaps between the census in the Hunter Valley, but that was okay, we were aggregating for that and accommodating it, but the inferencing was really tricky. And that's where, without giving too much away, the EPA offices were challenging the outcomes of this because it was, what they wanted to do, was take people who were money running organisations, who were creating pollution, they wanted to build a case to take to court to prosecute their air pollution.
But the inferences weren't giving them that. There was no way to understand what was causing the air pollution.
It was just literally counting particle matter. So there was a lot of disagreement around that and the usefulness of it which was the credibility went out the window too.
So that was a bit of a foul project.
But this is a nice way to kind of debug where things are breaking down with the data. And if that data isn't complete and it's not telling the right story or it's not doing its job, then they're going to have bad decisions being made on behalf of anyone else.
You're going to have wrongful judgments.
you're going to have people missing out on social services. Fortunately, medical and air travel, they've got this really down pat, so I think that there are industries to look for moving forward.
Where safety is really critical, but you know there's other harm obviously as well. There's psychological harm, there's social harm, not just medical and travel, physical safety. So if someone's interacting with their systems, they need to understand why decision's being made. They're allowed to know that, so this sort of the myth of the black box has got to stop. And there's a lot of good work coming out at the moment as well about how to explain the intention of an algorithm without giving up the commercial sensitivity of it. That's real and it's being looked at at the moment, so you know hiding IP around the kind of commercial sensitivity of an algorithm is actually bullshit. It can be, there's a way of auditing it and ensuring that it's intentions and it's outcomes are solid.
Is there a feeling of due process? So you should be oughta have a right of reply. If you're getting a, if you're getting.
I ran a workshop at DHS.
Someone who worked at DHS got a letter from DHS, and he didn't understand what was in the letter. And when he tried to go back to the department to find out in the organisation he worked for, they couldn't give him an answer.
Some system had spat out some kind of decision on his behalf and he couldn't get an answer from it. It's only days.
We'll get there.
Can they participate in their due process? Are you allowed to go back and challenge it? You should be allowed to.
And is there a feeling that the provider of that decision is owning it? Okay, it's like a brig's up there saying you know I'm sorry, I shouldn't have done it, but there's a whole bunch of other people in the organisation as well who should be held up accountable too.
And that can't just happen at the end of a big crisis. It needs to be happening the whole way through. And we're also seeing, so AI Now just released a great article, which I'll reference at the end here, it's designed to assist public agencies with explaining their algorithmic intent, right? So it means displaying what you're collecting data for and why you are collecting it and where it's going and what decisions are being made out of that. And I know Nathan's going to talk about this a bit more as well, so I don't want to go too much into that, but it's not presenting people with this huge, big contract that they have to agree to terms and conditions, it's you know releasing the right amount of information at the right point of time so people feel informed when they're interacting with technologies, they feel like they're being taken seriously, they understand what's going on, it's written in a language that they understand, and they feel like they're a part of our process. And this is basic UI design, basic interaction design, basic user experience design.
We're good at this.
And asking these questions: who and why? Who gets left behind? Who is being harmed? Who benefits? Informs a product strategy, the context of use definition, you know where that information needs to be surfaced and when.
We can adapt Neilsen's heuristics.
I'm going to you know make you suffer through that in a moment as well.
Inclusive design is really important.
This is where inclusive design really comes to the front. Everybody needs to be included.
Accessibility standards again.
And content design.
And I know great efforts are being made in our government and our government departments with digital standards around identifying this work and ensuring that government services are accessible and inclusive.
And so this is a really great start.
And content design, and I think we've got some content topic coming up as well.
So this pretty much if out UI's, we can adapt Neilsen's heuristics toward the UI's and it will go somewhere to sort of solving this problem. Visibility of the system.
Matching between the system and the real world. Using real language that people understand. Understanding where the data is in the system and at what point and what decision are being made with it. Supporting undo, right of reply, request an explanation of the algorithm and the data sources.
That should be made available.
You can get a copy of your own data as long as it's in line with the law.
You can withdraw your data, and we're seeing that happen now.
Facebook has finally figured out that you can actually delete your data.
That's very nice of them.
And you can edit or update your data.
That's in line with data privacy and access laws. So our online presences are actually are own to control. There are standards coming out now for ethical machine law. Learning and regulation is catching up.
Understanding where the trade-offs compete with standards and standardisation.
So sometimes you'd be able to use standard methods, other times you're gonna have to use customizable ones in sympathy with the work that you're doing. Remembering that every piece of work we do, we need to ask who and why not just have some sort of stamp of standards on it. Empathetic practises and inclusive design.
Error prevention especially around the uncertainty and predictions. So a lot of this with machine learning and large data sets that we're working with you can't just sort of say you know the traffic between here and the airport is going to be 90% crap today between three and four o'clock which I hope it's not because that's when I'm travelling, but you know give people, treat people like they're smart. Let them see that like the prediction that you're creating is not black and white. It's not like, we complain about the weather predictions, right? Because it was predicting rain? Well actually, they're not.
There would be a value, a range within that prediction of you know correctness as well.
And that needs to be explained so people understand that there's you know the machines are, they're not infallible. It's just data and rules producing an outcome, and there's a lot of variations in outcome. And if we explain that to people, then they'll feel more informed and more empowered about it. So whenever we want to describe the range of uncertainty and predictions.
90% chance of rain is like a 90% chance that there will be a 90% chance of rain.
And recognition rather than recall, so there's a lot, there's going to be a lot of information sitting in these technologies that people are ingesting.
So being mindful of that, again not sort of giving them some huge terms and conditions up front for them to read from top to bottom, but introducing the right information at the right point of time to help understand the implications of what's going on as they go through. Efficiency and flexibility.
You know if we're creating technologies where there are power users and we want to start building shortcuts in but the shortcuts might actually mask an issue in the data, then the system needs to be able to flag that there's an issue with those data sets. So for example, you might be a data scientist working with different you know different data sets, and you're trying to feature match those data sets, nine times out of ten the characteristics are the same, so you kind of got some sort of shortcut around that. But if the system notices that the schemas aren't matching, it should let you know because otherwise your outputs are going to be disastrously wrong. So these systems need to be aware enough to understand what normal operational use and if there is a shortcut or acceleration around that if someone is kind of setting up parameters and they use the same parameters every time, it just recognises that but it gives some sort of user control and freedom by alerting you to it afterward.
So that's true AI, right? It's not just sort of, I don't know.
Making good decisions on the fly.
The system, it's kind of like, the system is intelligent enough to know when it's wrong as well, and should let the user know so the human can get involved in correcting it. Aesthetic and minimalist design.
Again that comes back to content design.
Helping people recover from errors and helping documentation.
So I think the heuristics are actually quite, quite sound in this topic, and hopefully you agree with me.
So I'm kinda reaching the end here.
We're striving for ethical practises, not trying to have a clearly defined product. So the topic of values is a discussion that you need to have in your organisation. So if you're working for a betting company, that's one kind of, there'll be ethical values around that. If you're working for a government agency that has a large number of socially dependent recipients, you're going to hae a completely different set of values around that too.
So like ethics truly is about discussion of values, defining what is good, defining intent, understanding what the trade-offs are, and there's no cookie-cutter to this.
It's meant to be an ongoing chat with each other to work out what's going on and how you can progress it towards good outcomes. Reduction of harm.
Really important that diverse, multi-disciplinary teams are involved.
Those comments earlier about designers, I truly think that that's meant to be just more about technologists and hackers and the kind of software developers who are really, really happy building code, and they do a really good job of it, but it needs to be stat becoming a broader range of people who are involved in the outcomes that are being built. We can reframe our existing practises to do this work. And I think it's a good time to leverage that. And challenging "can we" with "should we".
Just because something can be made, it doesn't necessarily has to be made.
Not everything can be an experiment for fun. There are lives at risks, and that's really important. And identifying who those people are at risk, and bringing them to the front of the conversation is really key.
So as mentioned earlier, I started writing about this. I think the article's in pretty, needs work, so if you do go and look it up, forgive me. But at the bottom of that article, there's these references as well, so you can find that.
And I also need to thank my colleagues at Data 61, Lachlan McCalman, Bob Williamson, Guido, Ellen who is a consultant.
I want to thank Dr. Mathew Beard from the Ethics Society, Ethics Centre of Sydney, and also my team, Mitch, Principal Designer, Mitch, back there, and Liz and Phil who aren't here.
They've had a lot to say on this as well.
So we're working through this at the moment. Every day trying to find ways to sort of test these ideas and hopefully we'll come up with some sort of more extensible framework especially around machine learning, privacy preserving analytics, and public data use, but I would love everybody to kind of take a piece of it and go away and have a go, and let us know how you go.
Like get the community up and running, share your ideas, share your findings, share your patents, whatever.
I think that would be really awesome.
If you really want to know where to start, I would strongly suggest grabbing the IEEE Ethically Aligned Design report.
There's a short and long version.
And the AI Now Institute report, especially the one on algorithmic impact assessments. That's a really nice framework because it talks about regulatry, teams, social impacts as well in assessing algorithms. And I think that's it.
Thank you.
(audience claps) (upbeat music)