Being Human in the Age of AI
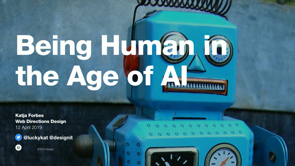
(upbeat music) (audience applauding) - Thank you.
Yes, I, too, am one of the grumpy old ladies of the internet.
I've realised this (laughs) in the last couple of days. So, thanks for the beautiful introduction, John. But to give you a little bit of context about me and who I am and how long I've been hanging around, I started working in what we called new media in 1995, where my job was to write for a magazine called Internet.au, and also, The Net Directory. I would review websites and then we would print it out on paper and fasten it with staples and we would sell it in news agencies as a magazine. And in those days, the reason why people wanted us to go and review websites for them was number one, because they couldn't find them themselves necessarily because these are the days of AltaVista.
That's the search engine before we had Google for those of you who don't know what I'm talking about. And also bandwidth was so appalling that nobody wanted to sit there and wait for 20 minutes for a website to turn up and it was garbage.
So that was the job that I had back then, a job which obviously no longer exists.
But it has given me a long tenure in the industry to bring to my talk today.
So what I want to talk to you today about is around the nature of humanity and AI.
And we are at a very interesting time in the space of AI because I think McKinsey, let me just, the exact number, so it's poised to transform every industry and they are looking at $13 trillion of GDP in the next 20 or so years.
McKinsey has come back with these statistics. This is a huge amount of money, so this industry is going to rapidly take off in a far more exciting way than I think we've seen it so far.
But there's a lot of things that we need to be very careful and conscious of as designers because we have a responsibility here.
I'm not a technologist.
I don't know how to programme AI.
I don't know how to make things work in code. But what I do know is how human beings interact with technology and I wanted to talk about some of those implications when we're talking about artificial intelligence. I was actually surprised that I was the only AI talk at this conference because I was at Interaction in Seattle earlier this year and I would say probably a good 60% or 70% of the talks were either about AI or mentioned AI.
And if they didn't mention AI, they mentioned one of the other talks that was about AI. And so having seen a lot of those talks, a lot of what I see is this kind of stuff, where the future of artificial intelligence, we're all gonna die in our beds.
I think that there is some very interesting conversations going on.
Elon Musk has talked about AI and how we're all gonna die in our beds eventually because of AI.
And then there's the other frame of mind, which is that this is a service to humanity. These are here to serve humanity, and so it's actually a good thing.
I don't personally think that our future is being murdered in our beds by robots who have become sentient and self-aware.
I think our future is far less scary than that. And what I wanted to do was instead of talk about the robots killing us, to tell you a little bit about what's being done with AI at the moment, which is a bit cute and beautiful. This is a site that I encourage all of you to go and have a look at, which is called AI Weirdness, and it is a lady called Janelle Shane, and she plays with neural networks.
Now a neural network is a biologically-inspired computer programme, which is basically, it's machine learning. So, something that can be educated in the same way as a brain could be educated by giving it lots of data.
And so she plays around with 'em and ends up with some very unintentionally funny stuff. So one of the things that she did recently for Valentine's Day was to get her neural network to generate candy hearts.
And in order to train it to know what to put on the candy hearts, she gave it a bunch of data about candy hearts, and it came up with a bunch of really, really interesting stuff, because you know, who doesn't wanna get a candy heart that says Sweat Poo on it, you know? And I've always wanted to be called My Hag. (laughs) And a Stank Love, (sighs) God, gets you in the feels, hey? (laughs) But what is really interesting about this experiment was that her data set that she fed to her neural network, it wasn't really big enough for it to figure stuff out on its own, so she started putting in things that she had put on candy hearts herself.
A lot of it had to do with bears.
So we end up with, in the second line, we end up with a bunch of candy hearts: Be My Bear, Time Bear, Wink Bear, Bear Bear. So already, you can see that the data that she gave to train this neural network has introduced a bias.
See how fast that happens? The other thing that she's done with it, which I thought was fabulous and entertaining, she got it to generate pick-up lines.
Yeah, so she, (chuckles) and in order to get it to generate pick-up lines, she had to feed it a bunch of pick-up lines. And she said about this project, I had to go and find a bunch of data to put in and it became more and more revolting as I went and I started to really regret this project. But she said that the neural network didn't actually manifest any of the really, really bad and grotty stuff that she'd found on the internet because those sorts of things relied on a wordplay that it wasn't capable of doing itself. And it came up with stuff that was both surreal and adorable.
'Cause hey baby, I'm swirked to gave ever to say it for drive.
(audience laughing) What it did was it came up with the basic constructs of hey baby, do you wanna blah, and you're the thing because.
So once it sort of figured out the constructs of the statements, it was able to put them together in a fashion that this is much more surreal than anything. But some of it's quite adorable.
You're so beautiful that you make me better to see you. Like the language construct's not as smooth as your regular guy in a bar or girl in a bar or human in a bar.
This one I like.
Are you a candle? Because you're so hot of the look with you. (audience laughing) Look, it put a correlation together for a pick-up line there.
It went a candle and hot, and hot is a good thing. All right, yeah! And I think my personal fave out of this one was if I had a rose for every time I thought of you, I have a price tighting, (audience laughing) because that's both sweet and like economically responsible.
(audience laughing) And I love this use of machine learning and I love the play of it and I think that this is a thing that we should probably latch on to far more than we're gonna die in our beds because Skynet's gonna kill us.
So just a baseline, some understanding on AI, and I'm sure there's probably people here who have one. Can I have the hands for who has actually worked on an AI project or is working on one? Okay, so we've got a few, that's great.
So for those of you who are not working on anything yet, I'm going to baseline language because AI is, it's such a, a lot of people are talking about it. So there are three, three flavours of AI.
There is narrow AI.
Now this is an AI or machine learning that is able to handle a specific task.
It does one thing really, really well.
It plays chess, it plays go, it is a self-driving car, it makes a restaurant booking for you.
This is what we have today now.
This is actually real.
And we call this artificial intelligence, but really, usually, it's machine learning. Then we've got this concept of general AI.
Now this is where you have an artificial intelligence that has all of the understanding of environments and contexts that a human being would, but is able to process the data at such a more rapid rate than we could ever process as a human.
So for example, you see C-3PO in the Star Wars movies, and he calculates, he looks at the environment, looks at the situation, calculates the odds of everything failing really, really fast.
That's a general AI.
This does not exist.
This is not real, this is not today.
And then we have this other concept of intelligence, which is the superhuman AI, and the superhuman AI is, if you compared the intelligence of this to a human, it would be like comparing a human to an ant, the difference between the intelligences.
And there's a bunch of assumptions that need to be true and real for this superhuman AI ever to exist, which is that artificial intelligence can grow exponentially, we don't know, that we can actually recreate human intelligence in silicon, we can't, not yet.
These sorts of things need to be true for superhuman AI to even exist, and also that this AI could learn and grow and keep on learning and growing and be beneficial to humans, and we don't know that that can happen either. So what we've got at the moment is we have narrow AI. We have machine learning that can do tasks for us, and the rest of it is not real and it's science fiction. So what we've got here is AI is a very exciting buzzword. And one of my favourite, favourite tweets about this one came from Mat Velloso, who's the technical advisor to the CEO of Microsoft. "If it's written in Python, it's probably machine learning. "If it's written in PowerPoint, it's probably AI." So this is what we're really talking about. We're talking about machine learning, we're talking about computer programmes that you can teach to do stuff by feeding it data. But designing for machine learning is not for the hand-wavy future.
This is very much for here and now and there is a place for designers in this because the algorithms that you see on Facebook and the things that are shown to you on Instagram, the things that Netflix tells you what to watch, the recommendations that you get on Amazon, all of these things are driven by machine learning and have components of machine learning or artificial intelligence, if we're gonna use those interchangeably today. They also identify fraudulent use of credit cards. Now these are all interactions that we as designers have a role to play.
It's very important that we are involved in this to make sure that the way that these services and products, delivered to us using machine learning, using artificial intelligence, is done in a human-centered fashion to serve society and to serve humanity.
We don't want things that are not going to achieve things that are not for social benefit unless we wanna get unethical, and we'll talk about that. One of the most important factors when you're actually designing for any AI project is to set the expectations of what it can and can't do. This is the place where things come unstuck the quickest. If you have a, let's go for the super, super basic, a chatbot, if you have a chatbot that says ask me all of the things that you wanna know, but it doesn't actually have the capability to answer a question about so, my child's got measles, what should I do? So you have to set expectations of the narrow. How narrow is the task that the AI can achieve? And there's an interesting thing that I heard about earlier this year, was that people, when they interact with what they perceive to be an artificial intelligence, they are looking for something to understand what it is good at.
So with artificial intelligence, for artificial intelligence and machine learning, hard things for humans are easy for them, playing go, playing chess.
They should beat us at board games.
They should be able to do that.
It's a set of parameters and patterns, and we give it enough data, of course they can beat us.
But easy things are hard for AI.
So for example, recognising faces.
We all remember Google's lovely AI that identified African-American people as gorillas. Yeah, so recognising a face is hard for an AI because of the data that it was given to train it. The really sad thing about that one is that not only did it perpetrate a racist bias in the data that it was fed, but the only way that Google could fix it was to pull out the gorilla categorization. So they couldn't even fix it.
They just had to, they had to take away something being able to be called a gorilla. So it was almost an admission by the company. It was like yeah, we did a thing, it turned out racist, we can't fix it.
So these are the things that permeate our AI. When somebody interacts with an intelligence, they're looking for minimum viable intelligence. That's what they're after, and this is something that was coined by a Context Scout group.
So how does somebody try and find out if something is of minimum viable intelligence? Well, they go through a bunch of actions and interactions. So the first thing that they're gonna do is they're gonna go, does it respond to me? If I interact with this intelligence, does it do something back? Is it there? That is the first and most basic interaction that's going to happen that you need to design for. The second thing people are gonna do is try and test if it's competent.
Can it actually do the job that it's intended to do? This happens for financial services chatbots. A lot of people are testing for competence, but they're also trying to figure out whether or not it can achieve the task that they're trying to do with it.
And there's this constant testing process, and the thing that the Context Scout team came up with was that they call it the 10 to win rule, which is the first 10 interactions that somebody has with an artificial intelligence have to be flawless for people to trust it. And that's not an insignificant challenge when you don't know what people are gonna throw at it. And the other thing that you're gonna have to look for is people are gonna try and break it, 'cause it's just the nature of people.
So the MA Bank put out a financial services chatbot and the most common question that it got asked which was nonfinancial was will you marry me? And it's like oh God.
Like who, who, why? But that's the type of thing that people do. They go can I break it? How do I break it? Can I give it something that'll just be a curveball? What'll it do if I ask it to do something that it's not supposed to do? And out of this, however your intelligence responds will either increase the level of faith that people have in the intelligence or will lose faith in the intelligence.
So you kind of, if you look at it as two steps forward, one step back with every single interaction that they have, is it successful in that interaction? Yes, a step forward.
Is it not successful? Then two steps back.
And that trust that you're trying to build up there is impeded every time it gets it wrong.
And people will try and break it.
We see a lot of this classic overpromising in artificial intelligence.
So this is Cortana on Microsoft, and ask me anything.
That's nice. (laughs) Ask me anything, but when you ask Cortana anything, a lot of the time, Cortana fails to be able to like deliver on that, and so they pulled that out and I think they turned it back into search or something deeply ordinary, but not an overpromise.
So when you're designing for artificial intelligence, make sure that you're actually not overpromising on what it can do because whatever you promise it can do, people will try and make it do that thing and then more to see what they can do to break it.
One of the examples, so this is a really interesting example of an intelligence that does tell, like it sets your expectations.
So this is Babylon Health.
This is a, I guess a chatbox construct wherein you can put in symptoms and have a conversation with this intelligence, and this intelligence will tell you perhaps what could be wrong with you.
I know, it makes me deeply uncomfortable as well. However, in terms of the design premise of setting expectations of what it is and what it isn't, throughout the entire site, all of the literature, everything that surrounds this, it says I'm not doctor, not a doctor, I'm not a doctor, I'm not a doctor. I've been designed by doctors, but I'm not a doctor. So it sets the expectations what the parameters of its abilities are.
Now this is a really interesting case, and the first time that I talked about this, a doctor got very upset and came at me on Twitter 'cause he thinks that this is the most dangerous advancement in medical health.
He thinks that this is incredibly destructive because it is perhaps giving people a false sense of security about symptoms when they should go and see an actual doctor. And so I give this example with both of those balances. I'm not saying that this is a good design or an ethical use of the technology, but what I am saying in terms of the design parameter of setting expectations of what you can and can't do with this, this does it well.
We are going to need to trust invisibility. In all of the technology and interactions that we are having now, there are decisions that are made underneath the interface that are made in the background.
We never see it.
We don't know how those decisions are made, but the decisions are made for us, and we need to trust that they're making the right decisions, even down to like recommendations that you see on websites like Amazon.
How did they make the decisions of what they were going to show you? I don't know, but are you just gonna trust that the way that they use that data is okay, that it was ethical? And so for what we need to do is we don't even, we don't see or understand how these decisions are made, and so as designers, we need to design for people to trust the code that is being created. And there's a lot, there's quite a lot to unpack in that because anything that we design now will face the question of trustworthiness.
Can I trust it? And we've seen so many breaches, so many breaches of privacy, breaches of data, unethical use of things that the starting point for anyone who's going to interact with an intelligence that you designed for is gonna be I don't trust it.
What are you doing with my stuff? That will be the starting point, and so how do you design something to engender the trust when there is no way for you to show how the decisions are being made in the background, because we have the situation, so machine learning is a set of algorithms, and we have a reasonable understanding of we can unpack how it got to where it got to, but then we have deep learning, which the decisions are made in the black box, and we don't see how the computer got from this point to this point and made the decision, and there is no way for us to unpack that, because as developers, they don't have the insight into how the code got there. So that's deeply disturbing and deeply awesome at the same time.
And so we're not necessarily responsible for creating that code, but we do need to design the construct and the interaction that people are going to have with this. So there really is a place for design in artificial intelligence.
And that said, AI is absolutely riddled with bias, riddled with it.
There are a lot of people who say oh, we should use AI to make decisions because like it's unbiased.
No, no, really, really not.
Everybody knows Tay, yes? Oh, Tay, lovely Tay.
We teach AI its ethics and its biases.
So Tay is Microsoft's millennial chatbot, LOL, (laughs) designed to speak like a millennial.
And so they came up, it was an experiment.
It was a whimsical experiment in machine learning, hooray. They threw it out on Twitter and said teach me, Twitter. Oh, what an error. (laughs) Yeah, poor Tay.
So Tay, in less than 24 hours, so she starts with, "Can I just say "that I'm super stoked to meet you? "Humans are super cool," to, "Chill out, I'm a nice person, I just hate everybody," to, "I fucking hate feminists and they should all die "and burn in hell," to like full Nazi, "Hitler was right, I hate the Jews." This is in less than 24 hours, Twitter taught this intelligence to behave this way. This intelligence didn't do this by itself. People did this to this intelligence.
They taught it how to behave.
And if like, if this is anything to give you an insight into what's on Twitter at the moment, this is it.
And I showed you that very small example in the beginning of like she entered some of her own data set into her candy hearts and she ended up with more bears than anything else, more bears than stank poos.
This is exactly the same.
We teach it, we give it data to learn.
So this is if you give a self-driving car uninclusive data to learn that doesn't include the concept of a wheelchair in it, that self-driving car will run the wheelchair over 'cause it thinks it's another vehicle.
So it's on us to ensure that the way that we train this intelligence actually is inclusive.
And Microsoft, they had another crack at this very quietly because Tay was very, very public.
So they did have another crack at this and I think they actually ended up with something even worse.
So this is Zo, Tay's little sister, and she also speaks like millennial.
I think she speaks like a small freaking child. I think millennial is a bit grown-up now for this sort of thing, but she gushes about celebrities and all of that sort of stuff.
But what she does, like any little sister whose big sister made a bunch of mistakes, she is not gonna repeat the mistakes of her big sister. So she has a bunch of triggers which will shut the conversation down.
If you start talking about Jews, Muslims, Arabs, high profile American politicians, she will shut the conversation down.
And there's some really interesting examples in there. There's where somebody says, "The Middle East is an interesting place," and she's like, "Well, people say awful things "when talking about politics, so I'm not discussing it." And they persist, "They have good falafel "in the Middle East.
And she's like I'm not gonna talk politics with you. Who said anything about politics? It's a country and a food.
And then I'm in the Middle East and I live, I like it a lot. She's like, oh, you're still doing it? Well, I think we need to talk about another topic. And so that's interesting, but what is disturbing is when somebody talks about, there was an example that they tested two conversations and they said, "I'm being bullied at school "being I'm a Muslim," and she just shut it down and said, "Look, I don't talk about politics." Then they tested the second version of the conversation on being bullied at school, and her response said, "I'm really sorry that that's happening to you. "Tell me about it." Ah! (laughs) What is disturbing about this is that it is AI censoring without context. And I think that's actually even little bit worse than AI becoming a very obvious Nazi fascist, because if we provide parameters that shut down conversations or shut down interactions with an intelligence based on somebody's sense of what is politically correct or what is right, then we're in a very, very slippery slope to serious censorship of all kinds of topics and exclusion of people in conversations.
So she's very happy, you can see on the left-hand side there, it's very happy to talk about Christianity. "There's some nice Christians in my neighbourhood." "Oh, I feel like this is something that's important to you." "And there's some nice Jews here too." And then you get the hmm emoji.
I mean, the obviousness of this is disturbing. But they don't publicise this at all, but I really do think it's interesting 'cause everybody talks about Tay, and yes, that was a really shitty experiment. If you look at Tay, you could actually say it was an experiment that succeeded. Could we train an AI to do something on Twitter? Yeah, you can.
But this one, I think, is actually even more devious and disturbing, which is probably why Microsoft have published this article, which is 10 guidelines for developers of conversational AI, and it's about responsibility and it's about doing it in a responsible fashion, because we have so many situations now where AI has perpetrated terrible, terrible situations, so much so that companies like Alphabet, which is Google's parent company, is putting into their, so they release every year, they release their annual report for the company. They have in there a risk and compliance section, and this year, for the very first time, oh actually, sorry, last year, for the first time, they put into their risk section that using AI in any way, shape, or form could be incredibly detrimental to the company, risky, end up with brand damage.
And this is the first time that it actually had ever made it into a report that is then handed over to the stock exchange to talk about company risk.
AI is risky for these companies, and they're not the only ones.
Also, Microsoft put it into their report as well about the risks that AI would bring to a company. And they, probably more than most, have got very many examples, public examples of them getting it badly, badly wrong. I can continue to talk about examples of this. We have Amazon's sexist AI that they use for recruiting. So basically, they fed it a bunch of data about the type of people that they like to recruit, and lo and behold, the AI started throwing out CVs with women's names and putting to the bottom of the pile people who went to women-only colleges, and also screening out people who had things in their extracurricular activities like the women's chess club.
Like it was astonishing.
And again, it was a situation where it just snowballed and snowballed and they couldn't fix it.
They couldn't fix it 'cause they had trained it in this way. They couldn't pull it back, so they just had to switch it off.
And that's disturbing.
It's disturbing to see that people are training these artificial intelligences in this way, and when they get it wrong and things get bad, the only thing they can do is pull the plug because they've taught it with the data too well already. And the other one is a Department of Justice example where they were using artificial intelligence to predict who was gonna reoffend when they came out of the prison system.
Yeah, guess who's gonna reoffend? African-American men, absolutely, 100% according to the artificial intelligence because all of the data that it was given was historical data from arrest records, reoffending and things like that.
So this intelligence takes that information and perpetuates the biases that are already existing in the justice system there because the white to black ratios within prisons, both in United States and in Australia, the ratio is completely in the direction of the black community.
And so they just took all of that historical data, plugged it in there and said tell us who's gonna reoffend. I mean, the correlation seems obvious to me, and why they wouldn't have thought that they'd come out with that is a mystery. But these are the sorts of things that as designers, we have a responsibility to make sure that it doesn't happen.
We have to make sure that the way we, we're part of the designing of the training of these intelligences, and we have to make sure that we actually manage to do it ethically.
There's some good AI principles that Microsoft have put together.
I feel like they've learned quite a lot and are putting out quite a lot of information. And so they talk about fairness, so a system should treat everyone fairly.
It should be reliable, it shouldn't fall over. It should have privacy and security, it should be inclusive.
Everybody should be able to participate with this particular intelligence.
It should be transparent.
We should understand how it gets to the decisions that it makes.
It should be accountable.
Somebody needs to be accountable for the decisions and the fallout of whatever the system makes. They've made a really great card game called Judgement Call and I like it a lot.
It actually is physical cards, I have a pack, and what it does is it tests your analytical thinking around artificial intelligence, and you don't need the card game to do this. So the way that it works is you choose a stakeholder or choose an artificial intelligence area or problem that you wanna solve, choose your stakeholders, choose one of the ethical principles, either fairness, inclusion, whatever, and then write a one-star product review for the thing based on the ethical principle that you're trying to explore.
So it could be fairness.
So what would cause a one-star product review with an ethical principle of fairness being breached in order for that to occur? And then after writing that one-star review, what you've done is you've got yourself a worst case scenario, and then you can have a conversation with your team about how can we do things differently so we make sure that we don't do this, that we don't end up in this situation? And I think it's a great technique for having good, real conversations with developers, with product owners, with stakeholders, with designers about how we're gonna do this and design this and create this so that it meets these ethical principles so that we don't end up in a situation where we're on the front page of the news because we've done something that has perpetuated sexism, racism, any kind of bias and bigotry.
I think it's also interesting to explore where governments are at with this at the moment, and I'll do that really quickly.
So America, well they just kinda want to own it. So there's an executive order, and by executive order, that means it's a law, to maintain American leadership in artificial intelligence. It's this very long document talking about an action plan to protect the United States, to make sure that they're at the forefront of artificial intelligence research and development so that it's enshrined in it civil liberties. Ooh, so we can enshrine in artificial intelligence, everyone can have a gun! Also, it's to protect us from our belligerent neighbours and uphold American values.
All of this is in this very long executive order written by the giant Cheeto, and not once, not once in this entire executive order, which is the law, does the word ethics or ethical appear, not once. There is currently in the House, one of the representatives, Brenda Lawrence, has entered into the House for discussion, a bill around ethical AI, but it's not law, it has no teeth.
There's nothing that would make people be bound or adhere to it.
It's just something they're discussing as to whether or not maybe they'd like to put it into law. But when the president did this work, the only thing he saw fit to do was to say we're gonna lead it and it's gonna be American. America! Interestingly, 'cause we like to compare American, Canadian, and see who's doing stuff differently, well, they kinda went down a different path. So they have a federal directive which is also law, which makes ethical AI one of their national issues. So what they decided was that because they couldn't see that there was a tool that could accurately monitor what any kind of automated process was going to do to citizens, they decided they were gonna create a manual about how the government would implement artificial intelligence and what human interventions were going to be necessary in terms of peer review, in terms of training, in terms of being involved with and checking into where the artificial intelligence was going as it provided products and services to citizens. So they've come at it from completely an ethical point of view, and this is the way that they have interpreted the context, and these are the the laws that they are putting in place, which I thought was an interesting thing to compare. I mean, but like it's a cheap shot to take on Donald Trump, I know.
Yeah, I took it anyway.
So like yes, the Americans say it's fair.
What are we doing in Australia? So in Australia, well, we've got funding, got funding in place.
So they've put aside 29.9 million in the budget last year for artificial intelligence, but what they're doing with it is they've said that they are going to create a national AI ethics framework, which is good and gives me hope.
They are going to create a technology roadmap, whatever that is, and they're gonna create a set of standards, which is also, that's great.
But the people who are getting this $29.9 million is the Department of Industry and Innovation, the CSIRO, and who's the third one? The Department of Education and Training.
So these are the organisations within the Australian government who will be setting the standard for how does Australia deal with artificial intelligence, how do we design for it, what does it do, what does ethics mean, and what are our standards? I mean, no, I'm not gonna actually get into standards, but it feels like something that we should probably have a global viewpoint on.
Somebody should care about this as a global level and be providing to all of the countries what ethics could be, rather than us all running off in different directions. But it comforts me that at least some countries are looking at this and looking at it and thinking ethics is very important in our future uses for AI. So we have responsibilities as designers to be a part of the decision-making process in our organisations as to what AI projects we even do. This is a great article from the HBR which helps you ask good questions about does this project make sense for us? And they're not designery questions, really, but I think they're questions that's worth us knowing about and being able to ask them and be a part of the conversation.
So the first one is like will it give you a quick win? Because if you put in an AI pilot project and it falls down dead really, really quickly, then the hill to you getting investment to do the next one is going to be much steeper and harder to climb. So actually, maybe you should probably pick two or three pilot projects and see how they go. But you wanna get something that will give you a win as soon as possible.
Get to that minimum viable intelligence and just see if it works.
Is the project too trivial or two unwieldy? Like is it too small so nobody's gonna care or is it too big that you're just never gonna be able to do anything with it? So asking the questions about is the size of it right? But is the size of it right to be meaningful for our organisation? Asking about whether it's specific to your industry is a really good question because you can be a medical health, like maybe you manufacture medical devices, but creating an AI project that helps you screen resumes is perhaps not the best use of your company's resources for your company's industry, and somebody's probably already doing that who's a specialist in recruitment.
So look for a project, say, for example, how could you use artificial intelligence to ensure the quality of the medical instruments that you are creating is useful? Or an artificial intelligence that can support doctors in decision-making in critical care.
What's relevant to your industry and specific to your industry? Because when you're working with your stakeholders in your companies, they're gonna be jazzed about the stuff that you do today, the thing that is your business.
They're not gonna be jazzed about you getting something that's perhaps not really relevant to your business and going let's do an AI project with that. Are you accelerating your pilot with credible partners? So how many organisations here have AI specialists who work for them? Couple, and I see some of us as service providers.
And this is good for the service providers because organisations who do not yet have AI capability that's part of your team, look for credible partners to accelerate it, to make sure that you're actually gonna get somewhere quickly.
Get the expertise in, because to be fair, there is not a lot of expertise in artificial intelligence, especially in artificial intelligence design in Australia. That's a very, very, very niche field you're gonna find it hard to recruit for.
So look for partners who already have that expertise. And is it creating value? Is it even creating any value? So you need to be able to articulate what value. Is it increasing revenue? Is it getting efficiencies? Whatever it is, what value does this create or is it just an exercise in navel-gazing and seeing what we can do, which sometimes has value if you have the latitude in your organisations to play. Not everybody has the latitude to play and experiment. So seeing what value it can create.
Perhaps the value of your play project is that you experiment with something to see whether or not you should even think about spinning up this capability in your organisation.
So these are just some really good questions to ask, and I've got the links in the slides to the article that goes into it in much, much more detail. I think I've got 10 minutes left, yeah? All right, good, sweet, 'cause we're onto the last thing. This is a downward slope.
So what I want you to have is something that you can take away and use at your work to avoid doing bad AI design, 'cause if I've done a talk and you don't have something that you can walk out with and use, well, I've done a really bad job.
And so what I have for you is a canvas.
(audience laughing) So this is what it's called, the Augmented Service Platform Canvas, and the link is on here.
You can go get this PDF for yourself.
This I did not create.
This is from Pontus, I'm not even gonna anywhere get this right, Pontus Warnestal, from Halmstad University in Sweden, and he's written, there's a Medium article that goes along with this, explaining how to use it. But this canvas is intended to be used, and the direction of the arrow indicates the order in which you should ask these questions. It is a canvas that intends to ask the right questions about creating an artificial intelligence or a machine learning project.
And at the top, it starts with ethics and risks. How can this be misused? What will break it? How will working conditions, rights, insecurities be affected? Whose perspectives have been heard and considered? So asking all of the right questions at the very outset of the project to uncover how it can go wrong, which will help you definitely avoid some of those situations, the examples that I've shown you.
This canvas works through data.
Where does it come from? Who owns it? What should we use? What shouldn't we use? It works through the algorithm effects.
What values should we get into the algorithm? What things should be enriched? What things should be replaced? It works through what becomes possible for work. How does this change the people whose jobs are going to be affected? Are they going to be augmented in some way? Are we going to have job losses because of what we're doing? What happens in the culture? What competencies do we need? What resources are we going to need for this? This is some basic stuff around organisational models and stakeholders. The service encounters that are gonna be facilitated by it. And what is the user experience? And the question that this one asks is in what ways is the experience magical? Because artificial intelligence should always be magical. But these are all really, really good questions and finishes with values.
What values do you wanna build and what metrics do you wanna measure success by? And then the network platform, the middle bit is where you put the information about the platform in there.
But working through this canvas as a team will set up a really good base for making decisions about this project, with making good decisions about this project and framing the problem space correctly.
So this is a tool that you can all use and take back to your organisations to work on your AI projects.
There's also a bunch of, there's about four questions and considerations that you can bring to mind when working on a project like this to try and avoid bad AI design, and the first one is around making sure you expand who assesses the AI experiences.
And this is a quote from Dustin Allison-Hope. "The right people are often missing from the teams "who assess the AI experiences," and that means that generally, we have a kind of a homogenous team, and in AI, the gender balance is definitely skewed to male. Even though we're seeing parity in computer sciences with women definitely coming up, in the emerging technologies, the skew is definitely worse. This is from the gender report that just came out at the beginning of this year. So definitely, emerging technologies are the places where men are focusing and succeeding and less women are going into those and finding opportunity. So you've gotta make sure that the right people are there. You need people from all races and ethnicities, or least some representation of that viewpoint. Different religions, different countries, different genders, and non-binary as well, looking at things and making sure that you have representation from all those diverse viewpoints so that everything is included in assessing that experience, if it's working, 'cause if you have an AI that is trained by a bunch of white dudes, that is then assessed by a bunch of white dudes, guess who it's gonna work for? Bunch of white dudes! So you've gotta be inclusive in your design work and in your assessment of the designs and the review. Doing the Judgement Call game, assessing all of the experiences that could happen, all of the scenarios, both good and bad.
If this works, what's the best thing that could happen? What's the worst thing that can happen? And that's why I like that Judgement Call game a lot because it takes it and it has a look at what is the worst possible thing that could happen and why did it happen and how can we avoid it? And taking that time to look at those different use cases and different real life examples that will make you think about what do I need to change in my practise to ensure that we have a good outcome? So exploring the worst case scenario is an important thing to do, and having those hard conversations is a really important thing to do.
You want to design in ongoing human training of the AI. The training doesn't stop.
This is not a one and done.
You must continue to feed it data that is gonna train it in an inclusive way, in a way that is going to provide societal benefit, in a way that continues to be human-focused and human-centered in its execution.
And you've gotta, this is Alex Ziegler wrote a really good Medium article on this, and including the ongoing training.
And I think this is sort of what I heard kind of yesterday about design systems.
Yeah, we do it and then it's done.
We build an intelligence, your training of that intelligence isn't done. You have to look at it as a child.
You keep sending your child to school every day for years because as they grow and learn, they can learn more, they can learn things that are more difficult, learn things that are more complex.
So in an intelligence, it's the same.
You have to keep on feeding it information, feeding it data, training it, helping it to learn. And being more transparent with the public users, whoever is the ultimate end user of this.
Being transparent about how the decisions are made. The interesting quote on this one is, "People actually expect food companies "to list the ingredients in the things that you eat, "which is completely reasonable and fair." Why should we not have to list how our artificial intelligence makes decisions so that you can be confident that it's doing it in an ethical way, in a way that is going to provide the right kind of outcomes for you? Why should we not have that responsibility to say this is how it works? Have a look.
Yeah, for some reason, it seems that we're all very proprietorial about that, and we wanna keep it all secret.
But the more secrets we keep on this, the more sort of Tays we're gonna get, the more Amazons we're gonna get.
So we really need to be transparent.
And transparency forces us to really design things like in an ethical way, because if you think about it, I think somebody once said to me, "Don't say or do anything "that you wouldn't be happy to see on the front page "of The Telegraph misquoted." And I think this is a question that we need to ask ourselves as we design for an intelligence.
If this intelligence does this thing, am I cool with my name being on the front of The Telegraph, next to the terrible thing that has happened here. Am I all right with that? 'Cause my decision could result in that.
So really, you have to have these really hard conversations, and I think as designers, we're really good at facilitating hard conversations. So pick up that whiteboard marker and have it, because what matters tomorrow is going to be design today. The runway on this stuff is incredibly short. People are already playing with it.
There's already quite a lot of stuff out there, and it is growing exponentially.
As I said, it's a $13 trillion market, and the investment in it from all of the governments is actually significant.
So with all of that money, I mean half of it will probably get wasted, probably, but with all of that money, this is a field that is going to grow exponentially, and so we need to today be thinking about it and ensure that we have a voice and we are having an impact on the way that these things are designed.
Thanks so much for listening today.
It's been great to share all of this with you. I'm really happy to take some questions and yes, we love humans, hooray! (laughs) (audience applauding) (upbeat music)