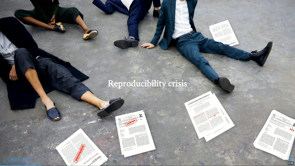
(upbeat music) (audience applauding) - Thanks so much.
I just want to share that I use talks as an opportunity to like, enjoy some weird creative time, so yeah, I have some very weird slides, and this is just because I was bored, but that's okay. Hopefully you'll find them entertaining.
So my partner tweeted a joke and I have to start the talk with this joke, because it's... Please laugh, it's really bad.
(laughing) So it's ironic that scientists now have the job of never reproducing the reproducibility crisis again. That wasn't, I didn't even tell it right.
We have to stop reproducing the reproducibility crisis. Anyway, I totally, it's better on Twitter.
Okay well, I've already like started off by embarrassing myself so we're good.
Okay, just to let you know, I have a lot of links. I've done a tonne of reading in this field and I've put a tonne of 'em at the end, so don't stress about capturing the URLs as I go through the talk.
But yeah, Ill put the slides at the end as well in case you want them.
So, lets get into it.
Our story starts with a TED Talk.
A social psychologist named Amy Cuddy gave a talk that was in, I think it was at the end of 2012, and it got an enormous amount of views, and it propelled her to a very specific kind of fame. So her talk was about this thing she called the power pose. So you can see her here, she's like the Wonder Woman. There's a few other poses, but her pitch was basically that by putting yourself in a powerful expansive pose, you can decrease cortisol, increase testosterone, become more powerful, and therefore improve your life.
So yeah, I know right? Thanks, thanks dad, I appreciate it.
So yeah, her claims are based on a field study that she had conducted.
She had a sample size of 42 people.
They did self reported claims of how they were feeling before and after the research, as well as saliva samples for cortisol and testosterone, and observed behaviours of gambling.
So basically they were invited to gamble or not, and these were the way she was measuring this. And the high power poses were expansive.
So they were like kind of powerful in the space, and the lower power poses were tucked in, and you know, maybe a little bit more timid. And the interesting thing is that, based on this TED Talk getting 51 million views, and just blowing up, a whole bunch of corporate people were like, power pose for the win.
And seriously like C-suite people and boardroom people were making a point of getting into power poses for two minutes before they would go into a serious negotiation or before they would go into an interview, and it really took off.
It really had this kind of big visibility, and people took it very seriously.
Now meanwhile at the University of Virginia, a bunch of scientists were asking this question, and I think it's important to note that this question was not new, but it had just bubbled up again. And that was are we doing enough work to double check our results? It's like that simple.
Are we actually confident that we're doing good research? And those questions were the springboard for the Reproducibility Project, which was led by Brian Nosek at the same university, and it was about 280 scientists who were attempting to reproduce 100 studies. So what happened when they tried to reproduce these studies is basically they couldn't.
There wasn't very good results.
They got pretty poor results showing the same kinds of effects that were observed in those original published studies.
And around that time, I think it was around 2013, 2014 after the reproducibility studies had been published, another scientist attempted to reproduce Amy Cuddy's work, and she did that with a sample size that was four times that original 42 sample, and perhaps unsurprisingly was not able to reproduce her work, and in fact everyone decided it was time to bandwagon and tear her down. So she had been raised up possibly without much consideration, and then just as quickly, everyone got onboard to tear down her work. And the thing that's fascinating about this particular example is that her work, this power pose thing became the flashpoint. It became the signal that everyone talks about as being the shorthand for flashy social psychological work that couldn't be replicated. So things that probably sounded like bad science before you even do the test, and then as soon as they get published, they get expanded and clickbait rewritten, and they kind of, the hyperbole increases in the curve, and we just absolutely can't really believe whatever it was they thought they were proving in the first place. And the reason I'm interested in this, is because I think the pressures we see on science are very familiar.
They feel a lot like the pressures we experience as UX researchers.
So I just wanna point out that I'm not trying to make us feel bad as UX researchers.
I believe in the value of UX research.
I think it's really powerful, and I think it's good to take inspiration from what's happening in science to hopefully protect ourselves from some of these same kinds of influences.
So in science, the problem is this thing called publish or perish.
So you either publish work in specific journals or you're a nobody.
And if you're a nobody, you don't get good jobs. You don't get tenure, you can't find a PhD position, or get a professorship.
So you publish and journals tend to privilege works that have unique findings, that have large effect sizes, and thing that are kind of interesting, right? So things that do turn into clickbait well. Also journals and academic institutions don't spend money on replication studies.
So the actual hard work of science which is double checking, doing it again, having someone else take a look at it, no one wants to fund that work, but that's actually at least half the battle. And yeah, as I said, clickbait is actually, it's silly to say it, but the ways that we take something small and simple and like conservative as a statement and blow it out of all proportion is part of the problem in science, because those pressures to create a statement, create a finding that can be you know, attention seeking can get people's eyeballs going. That's actually the opposite of good science. So P-hacking is a phenomenon that's observed in this space. I'm not gonna go too much into detail on stats, methods, and if you are a statistician, I apologise, but this is basically a graph that shows us something really interesting. So here you can see the black dots are publication studies, or sorry, published studies, and the triangles are replication studies.
They're trying to test the same things.
And you can see how these black dots are all clustering just on the underside of this line. That's because everything from here down is statistically significant. And this pattern, this plotting is not at all what we would expect to see, so this is basically human bias at large.
Like the fact that we're doing this, is us basically manipulating our experiments, and looking at different variables, and trying to find something that will eventually hit me under this line here, but it's not good science, and it's certainly not what we should expect to see. So when people say that quantitative research, or that numbers metrics based research is less biassed, just remember this slide, because it's really not. So yeah, I think we have similar problems in UX. We have bad incentives for doing the work.
Like prove me right, rubber stamp my idea.
I'm sure we've all heard this a million times. It's not a good reason to do research.
I've also heard this a lot recently.
People managers bending shortcuts wanting to get buy in into your results.
And it's like, if I'm halfway through the study, I really shouldn't be telling you what my results are. Also just this idea of like privileged hard data, like numbers based data over qualitative data, I think it's quite problematic.
And UX does have different goals to science, and it's important to recognise that.
Science is doing the work of chipping away at the crystal of knowledge, right? We want to know if something is true or not, and UX research is trying to reduce business risk. We're trying to give ourselves enough of a signal, enough of a warm sort of idea that we can say, right. We think we can give a crack, have a crack at this. But it's not the same thing, and it would be fair to say, well why do we care about reproducibility in UX research, and my answer is because we come from science. And Ill say this, we are science lite.
And I know that that sounds really mean, and I say it with love.
We are science lite.
Designed thinking, the double diamond, that is from science. Build, measure, learn, that is from science. So many of the methodologies that we use and talk about, and take as like a given that it works, they're all adopted and sort of like, you know jiggled a little bit, but essentially from the idea of the scientific method. So it's quite important for us to be able to go back and say, okay actually this is happening over here.
Maybe we can shift course a little bit based on what's going on.
So another thing I think is Important to keep in mind is that as ideas in the social sciences and social behaviours as John was talking about, get debunked, we can stay on top of that, and keep that in mind so that it doesn't leak into our research by accident. So I found this tweet thread and it's amazing. It's got a whole bunch of scientific ideas that are now debunked.
And the one I put up here on the right is one of my personal pet peeves, the Myers-Briggs personality test.
I know right? I'm not a fan of it because to me, it feels like astrology for people who should be too smart for astrology.
(laughing) And I say that because I don't want people to give me some random test and then say, oh, you're suited for leadership.
I get the same result as Margaret Thatcher and I really don't wanna be like Margaret Thatcher. Like I think, and I, look I don't, I know some people say that they got different results every time, but it just doesn't feel, it doesn't feel very sciency to me, and from what I've understood and read, it's not based on actual scientific research or any kind of empirical evidence.
So yeah, just keeping in mind what's changed, and knowing that can inform a research without us having to do any work is great.
Another common problem I'm sure you've all felt is this idea that people are using the wrong tool. They're using the wrong method to try and solve problems, and that makes the always hard problem of selling, internally selling user research even harder. So to make that real, I'm talking about people using usability testing to try and work out product market fit.
And it's never a good idea, and it happens all the time. So people saying, and I like this quote from Ha Phan in there. A lot of UX research looks to me like, well we gave users two hammers, and lo and behold they pounded nails, but pounded nails differently.
And it's like, sure, you asked a great question, you've got a great answer, but that's kind of the problem we're talking about, right? Like we have to get real about what we learn from different methods, and be articulate about that when we're doing the work.
As Paul was talking about, I'm not sure where he went, but as Paul was talking about before, like when we communicate the stories of what we're doing and why we're doing it, we have to get real. Like usability testing is to find out if users can use the tool.
It's not telling us if they will or if they want to. So, to get back to the question I posted the beginning of this talk, like do I think UX research should be reproducible, and this is just my answer.
Feel free to fight me about this later, but my answer is sometimes, it depends.
(laughing) And I think it depends on what we actually mean when we talk about reproducibility.
I've defined it as three levels, three layers. So the first layer is experiment design.
So what you actually are doing, and that's what model you are using.
Is it a survey, is it a diary study, is it user research, are you doing, sorry, interviews, are you doing ethnographic research, are you embedding in the user's contacts? The sample size: so how many people are you testing with, and how are you selecting them.
So do I know enough about your research that I could just do the same study again? The second layer of reproducibility is what data we capture. So is it going to look the same? If we ran that same study again, would we expect to capture the same kinds of results? And the third layer of reproducibility is interpretations. So if I then look at that same kind of data, would I expect to draw the same inferences from it? Would I expect to come to the same conclusions that a study earlier to me did? And Like, these are hard questions, and science doesn't get this right, so it's not just us, right? This is everybody's problem.
And when we talk about UX research, we also have this tension between qualitative and quantitative work.
So when we're doing qualitative work, I'd argue that the question of reproducibility can pretty much go out the window, right? We're not doing big sample sizes, and we're not focusing on trying to get lots of data that we can then look at in a more holistic way. So I think of qualitative work as like this work of art, right? We're taking someone's portrait.
We're drawing the picture of them.
We're understanding their story.
We're understanding their pain points.
When I did a test run of this talk, someone said, oh, is this the new user empathy map, and yeah, sure it is.
Take it away if you feel like it.
(laughing) And then quant is doing this work of looking at patterns, right? We're looking at behaviours, and movement over time. So yeah, my pitch is qualitative definitely experiment design, but you would never expect to capture the same data. If I did five interviews with five people, and then six months later five more interviews, I would in no way expect the data to be the same, and therefore I can't expect the interpretation to be the same.
And quantitative, again, like I would hope that the experiment design would be the same, and hopefully the data and the interpretation would be the same, but you know, like there are variables that can be hard to control for, and I'm not gonna say absolutely.
So that's the sort of sometimes it depends, but... So I've got a few pitches for you.
I think some of them are reasonably small and hopefully uncontroversial, and then I've got some big blue sky ideas, and I would love to invite you to talk to me about them after this.
So if you are doing the work of crunching numbers, and you want statistical significance, if you're doing like data science essentially, I'm only gonna give you two little pitches. One is make sure you practise this thing, research design hygiene.
Like make sure you get really articulate about what you're doing, what variables you're measuring.
Double check with a friend, triple check with another friend, and practise understanding the strength of your signal. And there are a tonne of resources that will do this for you, and I find it really helpful to take the same results from a study and plug them into different testing tools. I've put a bunch of links at the end of the slides if you wanna check them out.
But here you can see you've got your P-value and it's saying, yes I've got a really strong confidence that this is a statistically significant change, and it's showing me my confidence values here, but each of these tools does different things, and shows me this kind of data in a different way, and it can help you learn and boot up on stats. So if this is what you want to do, try other people's tools. See what they teach you about the world, and keep in mind that it's still not, it's still not gonna prevent you from doing totally stupid things.
So like, ooh sorry.
If you did your A-test in May, and your B-test in October, you've probably introduced a bunch of weird variables you didn't want, and you've probably invalidated your test.
And these tools aren't gonna prevent you from doing that, right? So you still have to use common sense, and try and do as much work as you can to keep the constraints of the test as clean as possible. But there's so many other methods we can use, and this is an old slide from the Nielsen Norman website, and I like to look at it just 'cause it reminds me that we're not doing new stuff, and these ideas have been floating around forever. And you can do research like studies, like what was the one that I liked, the usability lab study as a ethnographic field studies, yes.
Card sorting desirability studies, surveys, we can do all of these without necessarily looking for statistical significance, right? They can be in the qualitative field.
We're not capturing enough data to say we're looking for absolute certainty, but it can still teach us a tonne.
So other things we can do are try to embrace uncertainty like a scientist.
So that means we don't want to speak in absolutes. Science never says I absolutely know, or I don't know. It just says I'm working towards certainty. I'm kind of, I'm reasonably confident.
I have three other things that tell me this is pointing the right direction.
Cultivating your curiosity is great.
I like to whenever I have a feeling in my gut that's like, oh, I wanna know something, or I have a question, or something's unsettling me, I try and phrase it as a question to the team, like oh, does anyone else feel like there's something interesting going on here, or is there something we need to explore or unpack more. And rewarding I don't know is I think a big one. In the team, ironically the younger you are and the earlier you are in the team, the easier it is to say I don't know, but the more important it is, the further up the organisational tier you go. Like you find that people who are CEOs, and CSOs, and stuff really struggle to say this, and it's probably the most important that they say it because they make it okay for everyone else to say it.
So rewarding people at every level of the organisation for saying I don't know, so important.
Another idea is just defining your study before you start. So this is just an example of some research I was doing, and I don't know if you can read much of it, but this is just showing us, like is it qualitative or quantitative, what product life cycle phase am I in, is it early, is it exploratory, is it mature, what's the method I'm using, what's the question I'm asking, how am I capturing my sample size, and this is just enough discipline for someone else to look at my work and give me feedback, and just enough discipline for me to go back and remember what I was doing before.
Oh and just FYI, this is also something called preregistration in science. So people are actually registering the work that they're doing, and then putting it out in the world before they've done the study, and that lets other scientists give them feedback, and it's one of the tools that people are trying to use to avoid this P-hacking cognitive bias thing that we were seeing before. Another one I think is it's hard to talk about but important, is that sometimes what we will find out is that we didn't find anything out, and it's really uncomfortable, right? It's really hard to go to a leader, or a stakeholder, or an owner, like the highest paid person in the room, I forgot what that acronym was, a HIPPO yeah, and tell them sorry, you've just spent some money and what we've found out is that we didn't find out anything, but that's actually the nature of research. Sometimes it happens, and again in sciences, it's got a horrible name.
It's called we failed to reject the null hypothesis. (laughing) I know right, what does that even mean? But statistically we expect it to happen, and it's okay. And you may want to try and consider replication studies, especially if you have something that's important for the business to know over time.
So I was trying to think about what would be a good candidate for replication study in UX research, and I think it's something where we expect the effect to stay the same, or to be reasonably strong over time.
So that might be something like, say I sell cars. I expect people to want to keep buying cars, and if that changes, I'm in trouble, and I should want to know about it.
So that leads into the next idea is that, is this hypothesis or is this idea that we're exploring important to the business model actually functioning, because if it is, even if that does change, it's important for us to know it, so studying that thing again will help us, will make sure that we're on top of what's going on in our market, will give us little inklings of what's happening outside of our little huts. And conversely if it's lower risk, or if it's not a high priority to the business model functioning, maybe it doesn't matter if we don't know it. And another thing to keep in mind is, how much change has happened in your product since the last time you did it? I think that most products are in flux so much that you wouldn't expect the usability testing for instance to be a good candidate for replication studies because you're doing so many things in the meantime you'll never get a good apples to apples comparison.
It doesn't mean you don't wanna keep doing usability testing, it will just be a different study the next time. Another idea I had, and this is just a pitch, is that we might wanna try doing the synthesis and analysis part of our qualitative research with different groups of researchers.
So like you know, phone a friend style.
So here's my silly illustration of this.
You might have someone who captured the data, and she knows lots about it, and she probably already has a lot of opinions about what it means, and then she might say, hey, come in here, look at my big glob of data, do the same work of you know, trying to look for patterns, seeing what you think the inferences are from this data, and then comparing those two sets of results, and it might teach you something.
It might teach you that there are things that you learned in capturing the data that you didn't expose in the data.
Or it might teach you that there's some really obvious learnings, or next steps that everyone can agree on, and that will give you more confidence 'cause you've had two groups, or two sets of eyes looking at it.
So just a couple of power ups.
I think I'm doing okay on time.
Yeah, I had so much fun writing and making these slides. I think we can all get better as humans in the world, and you know, wannabe scientists at just fact checking, and that means if someone makes a claim about a study shows, go and have a look at the study.
And you don't necessarily have to read the whole study. You'll often find out what you need to know just from reading the abstract.
And I recognise that a lot of journals are behind pay gateways, and don't necessarily wanna spend a lot of money to look at papers that are of varying quality, so fair enough, but the abstract should at least give you a sniff test for whether it's on the right track. And I'd also like to pitch, please you know, if people are making terrible hyperbolic claims about science, maybe stop reading that news source. Don't reward them.
They're idiots, they need to go away.
And well I mean like morning news, I mean come on. Who needs morning news? Like they do so many terrible things with scientific papers. There's a John Oliver video I linked at the end of this, and they have a clip where they're talking, they're these morning news hosts, and they're saying, oh, there's so many studies these days, and they're all contradictory so I think the best way to do science is to pick the one that aligns with what you believe and go with that.
It's like, oh, come on.
So, another, kinda this is a big blue sky pitch. Tell me what you think.
Do we need open UX? Should we see open data, open results, like you know, FOS style.
Do we wanna open UX? Should we be doing meta studies across the UX research that people do that are now currently like all proprietal all inside corporations? Is that totally crazy? Does it scare the pants off of you, or do you like it? Right, like I think it could be really fascinating to see the research results that other people are doing. And also, I would love to get peer review from my friends. Oh my god, I would so love to have other people telling me their feedback about the research method, or how I'm trying to tackle finding out about a thing.
So that I think could be really fascinating. And then, again, similar to what Paul was saying, I have this idea that, this idea of doing a study, giving a result, and then moving on with our lives, is a bit of a missed opportunity.
Like we need to have a more longitudinal view of what we know, and what changes over time in businesses. So what would continuous discovery look like? How would we know what we thought when we started the business? What were the change points? Why did we pivot as a business? What did we learn, what happened? Could it be a timeline? Could I scrub through it and find out what was happening in the past? How amazing would that be for a new person coming into the team to learn something, to see the history of what the team thought they knew over time? So yeah, there's a product niche if anyone wants to work on it with me.
Talk to me later.
So to summarise my kinda more pragmatic recommendations, it's we wanna build our culture for that learning. We wanna make sure that we're actually asking questions that we're listening to the answers, and we're not just saying, tell me I'm right, let me do the thing I wanna do.
Embracing uncertainty: maybe considering replication studies if they're a good candidate. Obviously uncertain results are okay, but also getting comfortable with setting that expectation before you even start the work, and probably I think we could all push for a little bit more time in that design setup time, and the analysis like, sort of inference side. And I thought this was a nice summary of the vibe of all of these ideas, in short, be sceptical. Pick a good question and try and answer it in many ways. It takes many numbers or methods to get closer to the truth. Thanks very much. (audience applauding)
(upbeat music)