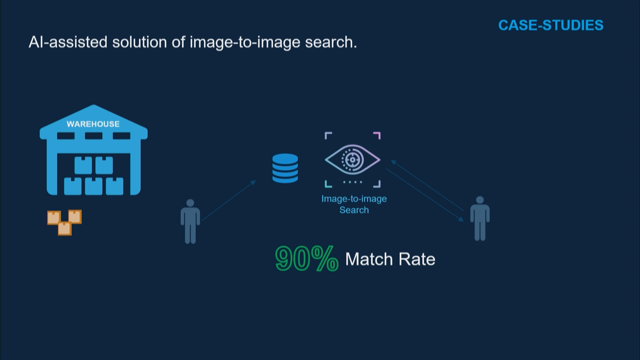
(electronic music) - I was actually outside at break and I was introducing myself and I said I'm one of the speakers this afternoon. And they said oh cool, what are you talking about? And I said enterprise AI and said, ah, the boring one. We'll try and make this interesting.
I live and breathe in the enterprise AI world. It is not the sexy AI that you hear about, it's not Siri, it's not Cortana, it's not Google Assistant and all that cool shiny stuff, autonomous cars. This is AI that's embedded deeply in some business process, making some process more efficient.
But these are some of the largest AI implementations in the country, in fact globally.
I've been in the enterprise AI space for a few years now and I've been part of a few very large scale implementations, large scale government implementations and stuff. I must tell you, I've seen more AI projects that have been unsuccessful than successful. This is from five years ago, six years ago. We were trying to understand what AI can do, again, back in the enterprise world.
So I'm privy to some extent on what's happening in this world of enterprises and how AI is being used there. What I want to achieve is, or at least what I wanted to achieve out of this session is if I can give you a little bit of a glimpse of what's happening in the enterprise world.
What I aim to cover is we'll quickly go through the state of AI in enterprise today.
It's constantly changing, it's rapidly moving, but I'll give you some quick numbers on where we are today. We'll talk about options for enterprises to consume AI and that'll go back to the question that you just raised with the previous speaker, in how can you go on a journey and adopt AI? Do you have to jump in straight away or is there a phased approach? So I'll cover that.
And then I've got three case studies.
If time permits, I'll go through these case studies. These are all real projects that we've implemented that I've been part of in some way for large enterprises. So I'll quickly go through them and then hopefully I can show you the efficiencies that we gained there and the productivity gains that happened through those projects.
I'll finish it off with the challenges where enterprises face in adopting AI.
There are few of them.
There's quite a few of them, but I'll highlight the main ones.
Okay, enterprise AI.
Just so I bring everyone on the same page, when I talk about enterprise AI, I mean AI that is used in the enterprise for enterprise decisioning or augmenting a human worker or assisting a process or making a process slightly better, making autonomous decisions in a business process. So that's what I mean by enterprise AI.
It's usually faceless.
You don't see it.
It's behind the scenes.
It's doing all the grunt work under covers. It primarily has these three components and many previous speakers have touched on these in various ways.
It has some capability to understand the environment. The environment in an enterprise comprises of documents, PDF files, Excel sheets, tables, images, videos, voice files, call centre recordings so we could process 20 years or 10 years worth of call centre recordings from the call centre department.
So that's the kind of environment this AI lives in and most of the solutions that we built have some sort of a capability to interpret and understand that kind of data.
And then most of the times it also has some capability to do some reasoning on top of it, so create a hypothesis and evaluate that hypothesis. Not all of the solutions have them, I must admit. Sometimes we want the reasoning to happen by the human experts or the subject matter experts, but all the understanding we let the AI do that. But irrespective of what AI projects we embark on, it always has a learning framework.
And that's what fundamentally differentiates an AI project from any other computing.
I mean, many of the techniques we use are standard computing, traditional computing techniques. But what sets AI apart is its ability to become better over time when it sees new data. All of the AI projects have the learning framework and different kinds of learning.
Paul touched on all types of learning, that was good, so I don't have to repeat that.
And so it's got some kind of learning that happens there. The state of AI in the enterprise.
I'm just sticking onto the boring theme on this slide alone. A few stats just to set some context.
I promise there's no more stats after this slide. AI investments, Jeremiah and his presentation spoke about AI in production.
He mentioned a very small percentage.
True, that's been the case so far, but as far as investments go, so this is the research conducted by Teradata, O'Reilly, and McKinsey together. Those 80 percent of the organisations in the enterprise world say they have some form of investment already set aside for AI projects. So that's a lot, so 2019 is going to be an interesting year. 2018 and before, yes we were just doing pilots and we were doing proof of concepts.
We were doing experiments and that was the appetite we had. We were trying to understand this technology. But 2019 is going to be a game changer.
All of these pilots that we've been part of are now going to go and see production, and there's lots of investments set aside.
The 42 percent of these respondents in that survey also believe that there is room for more, so they could spend a little more of investment aside for AI.
Another interesting stat is expected ROI.
Again from a previous talk, someone asked about what is the expectation? What's the return of interest on these AI projects? Some of these are expensive, big big spend projects. $1.23 in three years, that's the average expectation. That's what the research tells us.
$1.99 in about five years, and $2.87 in 10 years. So that's a very fair Auto I expectation for many emerging technology, for emerging disrupting technology.
That's a fair expectation now.
It never used to be the case but that's sort of getting aligned a little bit now.
And one last stat is 30 percent of the respondents in this survey, which primarily consisted of decision makers, C levels, the top leaders, they thought that that their organisation was not doing enough about AI projects.
They did not have anything in the next 12 to 24 months. So there were that as a bit of a concern.
That's it for the stats.
Not much, easy to digest.
Options, so I bring this slide up in most of my sessions just so we sort of know. AI is used quite loosely.
Depending on who you're talking to, they are talking about a certain AI implementation from their perspective, and it's quite confusing because someone said, you know what, my organisation just did some AI work.
What is the level of AI there versus me going and telling somebody else, I read this article, the Department of Defence is embarking on an AI experiment and you go yeah, I did that, too.
I experiment with that on my phone.
So there's various levels of AI that's at play. I've classified them into three categories. The first one is if you want to go and try out some AI capabilities, you could go and purchase a SaaS Solution.
These are usually available, they are turnkey, they're pre-packaged.
You can just go and bring them into your organisation and do what it's meant to do, so it's usually built to do just one thing. It's quick, but it's limited.
You have no control over the data sets.
It does what it is promising to do and that's all. You can't repurpose that to do anything else. But it's a good way to try what a probabilistic sort of a solution does to the enterprise as opposed to defined computing solution.
The next one, this I think is the most powerful one, is using cloud-based APIs.
Most big cloud providers have them on the cloud now. So if you take IBM, they have their Watson stack, Microsoft have their cognitive services stack, Google have their Google AI set of services. They primarily belong in vision, speech, and language understanding.
Prior speakers touched on each of these, so vision is object recognition, image recognition. Speech could be translation, transcribing, those kind of capabilities.
Language understanding is a very powerful tool again within the AI tool set.
And these APIs, the only problem with them is they enable rapid experimentation.
You can validate a concept.
You don't have to go and hire a data science team. You don't have to stand up a data science team. You don't have to bring engineers.
You can experiment with these.
But this is only going to take you so far, but it is enough to validate a concept.
The problem with these is they are usually trained on a generic data set.
So if you're looking at vision, it's really great at identifying cats and dogs, but bad cucumber and good cucumber need some training. We've trained generic machine APIs or vision APIs to identify machinery parts in a factory shop floor. That requires a little bit of training on top of these and they allow some training.
We call that domain adaptation, so we bring it and we adapt it to a certain domain.
You're still constrained in many ways.
You don't know how these algorithms work.
That's a good thing, many a times.
You don't want to know how the algorithms work. You don't want to know how the needle lead behind the image recognition works.
All you want to know is the recognition of the bad cucumber and the probability is just bad. It's not acceptable, so I'm gonna try something else. That is the kind of understanding you need. You don't need to understand full AI.
You can be a technical team collaborating with a business user and you can try these APIs straight away. The third option and this is the only way to realise full AI advantage, so this is when you bring in your data science team.
You stand up, you bring in your machine learning engineers, and you build custom algorithms and custom models. There's a range of frameworks available, from TensorFlow to Keras, Caffe, there's a whole raft of them.
And most of them, most of the good ones, thankfully, are opensource.
So there's nothing stopping you from going and installing this, provided you had the right capability, the right set of people, you can try with these straight away.
But it requires the organisation to have some level of AI maturity.
So when we go and do strategy workshops with organisations, we never advise them to start on building custom algorithms or custom machine learning models.
When I say custom, it's not purely custom.
You're still adopting an algorithm that was published recently, but it still requires a deep level of understanding of how these algorithms work. And that's when you need all that data science team and machine learning engineers.
So this will usually be after you've experimented with these cloud-based APIs.
And then you're comfortable that this solution will have some value and therefore, you want to go and spend the money and build a custom model. I'll keep going.
I'll keep going to my case studies.
So I've got three case studies, I'll see how many I can go. If it gets boring, give me some sort of a cue. I don't know, just look at your phones or something and I'll get it.
These are real projects I've been lucky enough to be part of and these were real successful projects.
I'm not talking about the other hundred unsuccessful projects that I was part of.
The first one is fighting fraud.
I think Paul touched on it as well.
So this was with a large bank, a significantly large bank. Ten billion dollars assets in management.
For any financial institution, fighting fraud is the number one priority, and so was the case for this bank as well.
They had a rules-based fraud detecting engine. So they had complex rules that went into this engine and it was working.
It's still working to some degree.
It was working fine for many years, but the landscape changed.
The environment now is different in the financial market. Financial transactions are much more connected. There's many devices trying to complete one single financial transaction.
So I start off on my phone and I finish off on my desktop my financial transaction.
That is a very complex nature of the situation now, and then there are ad hoc and there are anonymous transactions, so this rules engine-based fraud detector this was faltering.
This was not coping with today's scenario.
It was able to catch about 40 percent of fraudulent transactions, but the bigger problem was over 90 percent were false positives.
So it was detecting what it thought was fraudulent and it was not right, so it required a large workforce to actually go and verify that.
So it was just flagging off fraudulent transactions all the time, almost all the time.
There's not much efficiency in that.
So these were the problems, so they went on and they went on an AI journey, so they went step by step and we went through a three phase project with them. I'll talk about it a little later.
But they basically brought machine intelligence. They just sort of augmented their rules engine with some machine intelligence now.
And they were able to get 50 percent more at catching their fraudulent transactions and there was a reduction in false-positives as well, like 60 percent which sort of translated to lesser people involved to do the manual work.
The way they went about doing this, oh by the way, there was no additional data sets involved. It's the same data that they were dealing with anyway. We did not capture anything new.
It was just a better way of processing and extracting more of this data.
So the way they went about this is they went through three phases, and the first phase, again going back to the previous speaker, we sort of strengthened their existing analytics infrastructure.
We were prepping that infrastructure for autonomous decisioning, for automatic decision making. So we were getting the plumbing right.
We were getting the data aligned properly.
And then we introduced classic machine learning, so we took some features from this data and we created a model, and we deployed it. And we straight away saw efficiency gains.
But the game changing happened when we brought in deep learning technique, and quite bit has been spoken about deep learning. I'm not going to go into the details, but deep learning is a game changer, especially when you are dealing with larger amounts of information with large feature sets, lots of properties on this data.
Deep learning just makes a big difference.
So what the team did, the data scientists who were assigned on this project were tasked with finding the best machine learning architecture, let me put it that way, that suits the problem here, fraudulent transactions.
So we gave them the data sets and we said, what do you think works? Which of these machine learning architectures work? They went away and they did all the research, and they came and told us, actually, an architecture that's useful, image recognition just works so well over here. And we said, no, we're not dealing with images. This is text.
This is in spreadsheets.
This is tabular data.
I think many companies are trying this and many experiments are already going down this track. We took the raw data, we put it into a two dimensional matrix.
We were trying to make it look like an image, so we were getting it from rows and columns from tables and making it look like an image. And it did start to look like an image and once it started to look like an image, we were able to just look at this and eyeball fraudulent transactions versus non-fraudulent transactions or bonafide transactions. So the one with the red hue are fraudulent and the blue hue ones are bonafide transactions. So you don't have to look at the data.
Anyone can look at that and say, that is too red.
I'm gonna flag this off.
So we were able to convert text into an image-like form and then run the architecture that was meant to do image recognition or object-related stuff. But that is where we're heading.
I was talking to Nick as well, there's some deep learning experts in the room I know, that's where we're heading. We're trying to now treat text as images.
We're able to bring them in a form, an image-like form, because we have such beautifully developed algorithms that are processing image so well.
So if you can bring the text into an image form, you can just use these architectures.
So this is how we went about it.
Sorry for the details.
I'm not going to get into anymore technicalities there, but I'm gonna hang it on for a bit so I can talk about any of these projects until the cows come home.
Any detailed information, I can happily have a chat afterwards.
So this was the bank use case.
The next one is a logistics company that we did a project with.
So this was a typical logistic company.
They had a warehouse, packages would arrive from the shipper, get stored in the warehouse, and some kind of a distributor will collect these packages and set it off to the destination. Now, of these millions of transactions that happen, these distribution that happen, some would end up as orphan.
These packages would just be in the warehouse and no one there to claim them.
And the way they were handling these orphan packages was someone would receive a notification, a claim would be lodged, saying that that package is missing, it hasn't arrived at the destination. And someone would manually walk to the warehouse and look for that package based on description, based on labels, and whatever the case may be. So obviously they were spending thousands and thousands of dollars.
It required manual effort.
Absolutely pathetic customer sat.
I mean, if your package goes missing, it just means lots of phone calls, and it's just a poor experience.
So we did a very simple solution with these folks. We did what was called an image to an image search engine. What this solution did was if someone found out there was a package lost, they would send the picture of that package to this search engine, and the search engine would go through hundreds of images and say yes, I have a match.
And that database was actually a warehouse person, somebody who goes at the end of the day to the warehouse, takes a picture of all the orphan packages, and pushed it to the search engine.
And somebody could just self-help them.
It's a very simple solution.
It was just using image match, nothing fancy about it. The complexities and the size of image that we deal with, the enterprise architectures that we have to deal with, that's where the complexity comes.
But as a technique, it's a very simple technique. It's just an image to image.
90 percent match rate.
So 90 percent of the time, when somebody submits a picture of a lost package, if it's found in the warehouse, it says, okay, warehouse 25, found.
Then you go do what you have to do with that. Go and collect that package.
And the search window was from weeks to minutes. So that's another beautiful success story.
One more, you've got energy for another case, quickly? Yeah, okay, no one's nodding.
So this one was a New Zealand company, and they had a lease management software.
So they built a lease management software and it was apparently the most compliant commercial lease management software.
And therefore, a lot of the commercial leases were being migrated from the manual hard copy format into the software, and once it's on the software, it gets managed from there on.
So we went and met them, they had an unachievable target in front of them.
They had become so popular, and they had all the good checks, that all the little lease management companies were wanting to use their software.
And this company that built the software took upon onboarding these customers onto their software. There were 80 sort of part time-ish staff when we went there, and they were looking at these documents and inputting that into the software. It's data entry, but there was a little bit of skill involved.
These are legal documents.
It needs a certain skill to read a legal document and find out where the ABN is, and who the trustee is, and whatever goes in lease management domain. The problem was they had to get 60,000 customers onboarded in six months and they could've made the team bigger, but even then I think they were struggling. So again, I won't go into the technicalities of what we built, I can explain that later afterwards if anybody's interested.
But what we did was we automated the process, so we looked at all the possible OCR techniques that's out there because these documents were archaic. Some of them are from 1990s, poorly scanned, scanned that way and had noise on them, and you couldn't just digitise them.
So, we created a pipeline where these documents would go into that pipeline and come out digitised. And we were basically recreating the document in that pipeline.
So we would take literally a picture of the document, and we know there's a table there, and there's a signature there, and there's a seal there.
Pixel by pixel we would replicate that digitally. And when the letters and stuff were replicated, they became normal letters, digital letters that you can search upon.
You can say, control F, ABN, it would highlight all the ABN. So we were just digitising the documents.
A whole draught of techniques went into that. Let's not go into that, but stuff that people have already covered anyway.
A mix of image recognition, and a mix of digit recognition, and then language processing.
And they were able to do this with less staff, they were able to onboard things quickly.
But an interesting byproduct was because we processed these documents at this depth, they could not just search through documents, but across the entire document set.
So they could actually give a complex query, like show me all the leases that's managed in Oakland, that is in Oakland, which has two floors or more, and owned by more than one trust.
And such queries were impossible before and I say that 'cause that's 200 documents that matched the criteria.
And were able to just classify these documents, so you would just kinda say, okay, those are all the New Zealand ones, those are all the Queensland ones, and so on. So we could just classify them.
They could search on geography, they could search on time, they could search on date, they could search on semantics. And we were giving them never before insights. We would just go and tell them, hey, did you know that most of your properties that you're managing involves a trust and there at least four beneficiaries on an average on every trust that manages this? Things that they could not have imagined, so that was an interesting byproduct.
And now, they have a whole raft of opportunities in front of them on what they want to do with these insights.
They didn't come to us for that, but that was the byproduct. Those are the three use cases.
I'll finish off with the challenges and the barriers enterprises face to AI adoption.
The first one is strategy.
If you're embarking on an AI project, even if you're experimenting with cloud-based APIs, there must be a strategy in place, even before you touch any of the AI tools.
We spend a lot of time with our customers doing the strategy first.
So we bring them back and we say, okay hang on, let's see what the data looks like. Let's see what your vision is, and Jeremiah spoke about vision and stuff as well. So we ask them about what is it that you want to achieve? 'Cause you may be upset with what output you're gonna get out of this project, but are you okay to put that investment in today so that three years down the road, you have the value that comes out of this and therefore your future proved.
So AI usually touches across the organisation so a strategy has to be multidisciplinary.
It has to take into account not just the data and models, but the people, the culture, and of course the revenue and the financial elements.
You have to have executive buy-in.
This is where we struggle.
It's not the executive's fault.
Most of the times I'm sitting in boardrooms with decision makers.
They have very unreasonable expectations out of AI. That's because of all the vendor hype that happened in the last year or two years. They come with the notion that AI can solve world hunger, AI can solve poverty and many a times they ask me, is that all you have? Tell me you've got more.
And I go, nope.
That's all I think we can do with this.
And they go, are you sure? I think you're not good enough.
I'm gonna go and talk to somebody else.
That's the problem.
Either that, or the executives and the decision makers are not informed enough to make a decision, so they just push the decision away for later. Education is very important.
Someone touched on that as well.
Education is really important at the right level of complexity.
You don't want to be teaching reinforcement learning or transfer learning to an executive in a boardroom that they're gonna put off.
They'll say, I knew this was gonna be complex and that's why I'm not gonna go on this AI project. But to a level where we can align the expectations, this is what AI can do today, and here's your three year road map and here's what three years later what we think this platform can evolve into. The next challenge is tech.
This is not new tech.
This is completely new tech from all aspects. To start with, the frameworks, the machine learning frameworks are all opensource like I told you, TensorFlow for example.
There's lots of good things about opensource, the whole community coming together, and there's rapid developments happening in opensource. But there are challenges and problems with opensource. It's known for poor interoperability.
There's no one accountable.
Which phone number do you call when TensorFlow doesn't work? That's the problem and that is indeed a barrier, so that sort of puts off people, but I think we have to work around that.
My thinking is that opensource is gonna dominate the AI scene next year and even the year beyond that, so we have to come to terms with these powerful opensource platforms.
And then the cord and little pieces of artefacts that we find is usually academic-grade.
When I say academic-grade, it was experimented in some lab in a university as part of a machine learning course.
That is very different to enterprise grade or production grade things.
These are theirs.
Someone said why on Earth would you go and build that algorithm? You can just download that from GitHub.
I've seen that before.
Yes, but we can't just deploy that.
That wouldn't scale.
I can't put on a highly available platform, it's gonna break.
It can't handle two million transactions in a minute. So there are issues with what is out there. There's good stuff out there, but it still needs some kind of prepping and some kind of massaging before it comes to the enterprise grade.
And then you're working with specialised hardware. You all know that by now, it's not the typical hardware that we used to use.
Most of the customers that we go and talk to have to procure new hardware or infrastructure. It could be a cloud-based infrastructure, but they have to nevertheless go and procure new stuff. GPU based computing, I was talking to somebody, or TPU based computing, no one has that.
They never needed that before, so that's a little bit of a barrier as well.
These are all from our experience of going and dealing with these customers in person, so these are real problems that we face.
Data is a big challenge, again.
Data is also an accelerator for AI.
AI is where it is today because of data.
It's just the abundance and the availability of data, there's so much data today, that we're able to train models that we could not have trained 10 years ago. Having said that, that data is not in a format ready enough to be trained, that's what somebody said. You spend three fourth of your budget getting the data right, I think you mentioned that, Tim. You spend three fourth of your budget getting the data and the plumbing right.
That is the case, so the data has to be labelled, for example, in supervised learning.
And labelling is usually a tedious, tedious, very tedious human task.
You need lots and lots of minions to go and say that cat, that bad cucumber, that's a good cucumber. You have to go through that, that painful, tedious process.
And the last one is talent, of course.
Talent is an accelerator again, there's lots of people going to university and studying AI, but the problem is they are in the universities. We need industry professionals, domain experts with a bit of understanding of AI. So that's gonna take some time.
I know of many people, at least in my network, where they are making techies, making a pivot, making a career change and they're experienced techies getting into machine learning and getting into data science, so hopefully when that cycle happens, you'd have very experienced industry folks with also an understanding of how machine learning works and the under covers, these technologies work. That's it, wow.
That was abrupt. (laughing) But questions, those are my social handles if you want to get in touch with me.
- [Host] That sounds good, thank you.
- [Dheeren] Thank you. (audience applauding)
(electronic music)