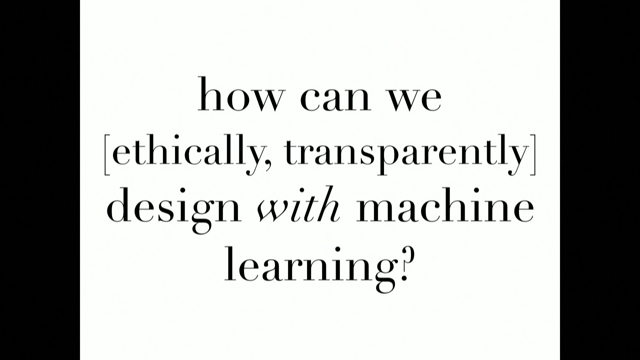
(upbeat music) - Hi everyone, I'm Caroline Sinders.
I am a design researcher, the founder of Convocation Design and Research, as well as a fellow with Harvard's School of-- Sorry, it's early, I'm still a little jet lagged. Harvard's Kennedy School of government and policy as well as a writer in residence with Google's Pair People in Artificial Intelligence Group. Effectively that's the group that's designed into Project Cerebra to look at ethical uses of machine learning.
And I wanna talk to you about some work that I've been doing and I actually wrote a brand new talk for web directions so I'm gonna be reading from some notes but this is one of my favourite conferences so I'm really honoured to be here.
Hi I study people on the internet.
I've been studying people as I would like to say as a sort of digital anthropologist for the past eight years.
I have a Master's from NYU's Interactive Telecommunications Programme.
I went there specifically to work with Clay Shirky to look at how people are using and misusing the internet.
That led me to looking a lot at cases like online harassment specifically and social networks. What's fascinating about online harassment is all online harassment are data points.
So if you're interacting in any kind of system with a conversation it's a data point. And what's really fascinating is that there's a space now that's trying to look at online harassment through the lens of machine learning, which is a really interesting space to explore. That's sort of what led me to pursuing a fellowship with Buzzfeed.
Looking at online harassment and machine learning and what were the ethical constraints that would pop up. What would be the ethical problems? This was really sort of the foundation of my work. And that's really led me to thinking about as a UX designer, cause that's really what my practise is in is UX and design research.
How can we design with machine learning? But how can we specifically design transparently or design ethically alongside machine learning? How do we communicate this to consumers? How do we communicate this to users? How do we think about actually what the product is you're working? Products- the product interfaces I think are the spaces in which users most engage with, whatever you're working on, right? But before we even get there I wanna cover some, perhaps, well-known stumbles with machine learning, specifically in how weaponized certain products can become even when they aren't intended to be weaponized.
And these use cases are really coming from two categories I've noticed from how users respond to different kinds of machine learning, specifically from a product design stand point. And that's one thing I want to highlight: if something can be purchased it's a product, right? So any kind of software capability you're creating, if it can be purchased I would advocate thinking of it as a product.
Which means thinking about all the ways in which it could possibly go wrong, interacting with an entire consumer/user base. Not just the ways you've intended it to be used, not the specific perhaps design intention of your natural image processing API but what are the ways it can actually go wrong when it's sort of been out in the wild? And I want to pick one example that's close to home. Sorry I should also mention, So I've noticed these two categories, one is Intentional Adversarial Algorithms another one is Unintentional Adversarial Algorithms. Pun intended with adversarial there.
So I'm from New Orleans, Louisiana and what was really fascinating over the past year is that New Orleans actually privately partnered with Palantir to create a kind of system to analyse different use cases around gang violence.
What's really fascinating is that this relationship is really hidden from civic and civil servants inside of the local government.
No one was really aware of what this relationship looked like because it came under a bigger relationship under the Rockefeller Center's 100 Cities of Resilience.
But what was really fascinating is that, when this was exposed through live investigative journalism first done by Vox and then picked up by the local newspaper The Times Picayune, the city decided to quietly step away from this relationship.
Because what had happened was, it was exposed how New Orleans what using technology, specifically artificial intelligence systems inside of a civic area.
But what they were doing was they were using it specifically around policing.
And there are plenty of examples that have existed in the United States, most recently done by the investigative journalist site ProPublica on the problems of trying to use artificial intelligence predictive policing. I'm pretty sure I'm not talking to anyone here maybe working on maybe DOD contracts, that's the Department of Defence, or British policing.
But it's important to highlight that this kind of product that Palantir had built for New Orleans was intentionally designed around predictive policing. It was a product that was built to analyse data sets and relational data sets.
Well what's important to highlight here and I say this as someone from New Orleans is New Orleans, Louisiana sits in this specific part of the south of the United States.
It's an area that is historically racist.
It's historically historically racist, building up from the civil war.
So a kind of day that exists in this area actually is incredibly biassed.
Black neighbourhoods in New Orleans are overly policed and they're overly policed in a specifically biassed kind of way.
So what's important here is not just the intentional adversarial design of the system, but the way the system reacts with data.
In this case specifically historically biassed data. And more importantly that this relationship was actually really hidden from civil servants to be able to provide any kind of checks or balances. Another example is Faception in Israeli face detection programme being used to detect emotions in faces.
Now the problem actually with this, other than the surveillance aspect, is how wrong these things can be and when we start to think about the emergence of emotional analysis among faces.
How many of you have heard of resting bitch face? (laughter) Okay so, you can look or be extremely happy. One of my best friends Laila has resting bitch face by then how she emotes.
So she'll text with a lot of emojis such as I'm so excited to see you but in person, I've missed you so much.
There's not a lot of emotion in her face so any kind of emotional analysis inside of facial recognition software would be misidentifying Laila.
Or perhaps another more heightened example, how many of you have ever felt uncomfortable but had to smile in an interaction? I'm sure a lot of different marginalised groups in this room know what I'm talking about. Well are you happy in that moment? You probably aren't.
But you're performing what looks like happiness. So this also brings up a deeper question of one, was the data set? Two, was it trained on? And three, what does it mean to emote publicly in spaces? Are you always emoting publicly the entire time? Or are you emoting exactly the way you feel? But this is even more fascinating if we start to look at predictive policing as a form of product design inside of artificial intelligence.
Blaise Aguera y Arcas had a Google's Machine Learning Group wrote on medium that predictive policing was listed as Time magazine's 50 Best Inventions of 2011 as an early example of biassed feedback loop.
The idea is to use machine learning to allocate police resources to likely crime spots.
Believing in machine learning as objectivity, several U.S. states implemented this policing approach. Half or many knows that the system was learning from previous data.
As I've said earlier, as police were patrolling black neighbourhoods more than white neighbourhoods this would lead to more arrests of black people.
The system would learn that arrests are more likely in black neighbourhoods, leading to the enforcement of the original human bias. But these two examples are really heightened, they're really specific.
They are intentionally adversarial designed products. They're designed specifically around police mitigation we could argue.
Or any kind of space where you're thinking about crown control, et cetera.
But what about Unintentional Adversarial Algorithms? What about products that have just gone slightly awry? So I should have a line down the middle of this slide and I don't and that's my fault, but on the, I guess it's by my left your right is one data set, it's one google query and this is another.
And does anyone have any idea what those queries were? One is professional hair and one is unprofessional hair. I know, it's pretty bad.
And if you were to try this out today, Google did course-correct after a lot of negative press. But it's really important to highlight this. I don't think the engineer, or the team that set out to create specific kind of image search queries on Google search decided to make something that was racist.
I don't think that's anyone's intention.
But that doesn't lessen the harm of this kind of output, right? Which sort of leads us to the deeper questions of what was in that data set? How was this query tested? How was it QA'd? What did the team look like? What were the series of questions that people went through when they were rolling this out? Google search is a product.
It's not just a technical capability.
It's a product because people use it, and they use it in their everyday lives.
I think this is a really great example of thinking about how something that seems to be purely technical infrastructure actually exists as a product and the kind of unintended harm and consequences it can have.
Another example I wanna give also around Google search is Professor Latoya Sweeney's work.
She is a professor at Harvard.
Professor Sweeney started working on bias and algorithms in 2013.
She started Googling 'black American-sounding names.' And what she noticed was that these triggered more ads related to arrests than other names. She googled her own name and the ad 'Latoya Sweeney arrested? Bail Bonds' popped up. Professor Sweeney has never been arrested.
This is just another example again of thinking about just trying to reinforce this idea of again, Who QA'd the product? How are you thinking about all the different ways in which what you are making could possibly go wrong? This is another example.
I guess the sound's not working.
What we have are two employees in a computer store and camera store trying out face tracking software on HP's laptop.
It recognises the white employee but it doesn't recognise the black employee's face. And again I don't think people set out to design a racist product but it does really again, bring up the question of What kind of QA process went into this? What kind of data sets were used? What did the engineering team look like? Right? What were all the processes to rolling this out? Cause this is a product.
It's a product embedded in a bigger product. But it's important to think about this capability again as simply a product and not just, We got it to work, right? We're using this really amazing system.
It's face tracking and it's recognising a variety of different faces.
I'm sort of showing this again to build up this bigger example of what is data? And what are the different kinds of data sets you're using? I'm gonna switch a little bit back over into some of my previous work.
So conversations including interactions, gifs, and likes are data inside of digital systems. So that means that all data we're kind of dealing with, especially when we're looking at human interaction is our human outputs.
That means anything again like online harassment is data but it's data about a person's personal traumatic experiences.
So I like to sort of show this at a high level of what I'm thinking about the way you break down the idea of data into smaller buckets and I'm sure for this audience this is probably a little too 101 but it's one I like to show in general, just when you think about weather for example, what are all the different kinds of things that can exist in the data set of weather when you start to create sub-topics and then more sub-topics and more sub-topics? And it's important because data is I think one of the key ingredients for machine learning and artificial intelligence because data can help determine what an algorithm really does.
So with the rise of machine learning being used as technical infrastructure and large products and process used in effect, almost every aspect of our daily lives, I think it's important to start touching on this notion of "transparency." So transparency has become this wildly popular concept to help define I think ethical parameters and suggestions for making, using and implementing machine learning.
And data gets involved in this.
What data was used? What's inside of the model? How big was the data set? And this is important because we need to start thinking about algorithms as a product selling point.
Throughout this presentation I'll be referring to infrastructure as being the skeleton or outline or the components of an app, a product processing service, or idea and machine learning is the skeleton around that.
Algorithmic suggestions of a music playlist or suggested movies, the suggestion in this example is the product. That's the algorithm at work.
It's the selling point or the reason for existence for that playlist.
It's not actually the playlist itself.
And the algorithm is now the process of how that suggestion is made.
It's activated in a way and it's using user data from listening, likes, interactions and replays. This idea of activated data combined with algorithms is incredibly important.
Bearing this in mind, what does it mean to call for transparency inside of machine learning? More importantly, what does it mean to actually design for transparency? Transparency can mean many different things to many different people and it can be used or weaponized for marketing campaigns or as even a plutonic ideal of safety.
Or it can be something that we have to implement for users to better relate to our products. So actually what I wanna break down is when we talk about transparency are we really talking about trust? And are there two different forms of trust? And I think perhaps more broadly there are Implicit Trust vs Explicit Trust.
So trust is deployed I think as a very modern design trope that really should describe an ideal relationship between a user and the product itself.
What does it mean to design for trust? We can start to look at things like GDPR for example. So it's important to highlight that all trust actually could be gained.
But what's key here is how trust is articulated and shown to users explicitly through your product design.
Explicit trust is seemingly clear and seemingly transparent because an action or a policy seems to be clearly stated and this is where the GDPR examples I think are really important.
And I'll actually be showing a lot of things outside of machine learning to sort of build up this thesis I have around what does it mean to design for transparency in machine learning. So privacy policies are another great example. Though privacy policies can be confusing, and the GDPR notifications for example can force consent cause you really can't opt out of them.
You can just select them.
I don't know how many of you have gone through a variety of all of your websites just doing all of these different pop ups of 'by the way, we're tracking you.' But you really can't say no.
But you have to keep reading this website.
But the point of this is that there are actually very clear statements of what is happening which can feel explicit.
In her 2016 book Calm Technology, Amber Case highlights how privacy policies can help create systems of trust in a product. Great user privacy experiences mean that your users will understand the privacy policy you've created when they start to use your app. A well-built app will let users know that they're opting into when they're using your software case rights. Design can be the case to show these policies such as designing opt in UI and user flows. Case continues by describing how to explain privacy policies to users by using plain language and offering multiple opt-out points such as explaining what data product service is collecting and why, how that data will be used, allowing users to download their own data from the product, allowing for the option to permanently delete accounts and remove data from the company servers.
Case argues that these design considerations are a form of transparency and that this form of transparency creates user trust with the company.
In fact, we could argue that that's true.
Case isn't just describing well articulated privacy policies.
What she's actually doing is advocating for user agency inside of products.
The opt-out isn't necessarily a form of transparency but an interaction where a user can take or have some control over how they're interacting with the system.
The user gets multiple choices in this case and not one specific user flow or interaction to be forced into.
So the showing of data used and describing what is done with it are key I think in building concise and explicit trust designed UI specifically for machine learning. And I do think it can be a form of transparency. But what if you can't necessarily show the data sets you're working with? What if you can't necessarily talk about data, right? And are there other forms of trust that can obfuscate transparency? This is where implicit trust starts to obscure notions of transparency.
Implicit trust is not directly stated but implied. The examples that come to mind are large companies using data to often engage in acts of explicit trust without showing how the data is used and why. That can be pretty much any product from Google. It can be cookie tracking or perhaps more adversarial, it can be how Cambridge Analytica used Facebook's ad-tracking system.
But there are times in which you need to be what I call transparently opaque and really using implicit trust with your user base. So a couple years ago I worked as an online harassment researcher for the Wikimedia Foundation that's the non-profit that powers Wikipedia, donate to Wikipedia.
And my research had to be open source and it had to be shared with the community and that's actually a major stipulation of the Wikimedia Foundation.
But already you can see the problem with creating open source research about online harassment. Because if every data point of online harassment someone's personal trauma, should you be sharing that? And you shouldn't be.
But what I realised is I could create a system of transparent opaqueness.
So I could remove specific kinds of user data that could 'out' a user like location, name, gender, et cetera.
What we created with my team was a form of semi-public research methodology called being transparently opaque.
So we would never specifically show or say 'we pulled these cases from this specific Wikipedia page.' Instead we would speak really generally such as we ran three surveys over the course of x month that broadly x number of individuals filled out. Then individually I spoke to between this many users over this kind of time and date from this variety of locations.
And in this case, we also looked at these general pages. And then from there if we had to share a takeaway we made sure it was completely anonymized.
And in this way we were engaging in the sort of transparent opaque research but also trying to build upon this idea of implicit trust that we were sharing as much as we could with the community while also explaining why we couldn't share everything.
So I'd like to argue that trust actually is not transparency but it's a byproduct of transparency which leads us back to my original question: What does it mean to design for transparency and how do we do this specifically in machine learning when you're potentially using sensitive data? Data, especially machine learning, for all digital products is important.
Data comes from people.
Which leads me to these three different steps I've created for how I think about designing for transparency.
So transparency is a mixture of legibility, which is the ability to understand, auditability, which a word I made up, the ability to understand a process, data point, or intention which builds upon legibility. Meaning you understand what's outlined clearly enough that you can have an opinion on it and you can know when something's going wrong. And then building upon that is interaction or agency: the ability to affect change or decision making from legibility and auditability.
So legibility is stated or revealed to us but the process should be understandable.
So let's examine bureaucratic procedures in this case. In this example the process user revealed, if you've ever interacted with any kind of government programme, there are clear steps you can follow.
That doesn't mean that you actually understand what the steps are.
If any of you have ever read any kind of bureaucratic language you probably know what I'm talking about.
It can be incredibly confusing.
And the key takeaway here is important.
You can share or say what a policy is but it has to be understandable for it to actually be a legible process.
So what is legibility in action in design actually look like? So the design in this case is not just UX, UI, or graphic design.
It's distilling or rather translating complexities into an artefact.
That artefact can be an app or it can be clear and concise documentation.
18F, a design and technology firm centred in the arm of the US federal government, designed a content guide and curriculum on how to use 'plain language' in governmental and federal services.
What they created was actually as asset.
It's flexible in that they were guidelines designed to scale across governmental procedures, designed also to serve as a dictionary but as well as a general guidebook.
Which means it could scale this idea of legibility across something as large as the United States government.
And this is an example of legibility not in practise. This is a UI popup that appeared on phones inside of the state of Hawaii where a governmental employee accidentally sent out an emergency alert that was not true.
This caused a lot of harm.
So legibility is important when it's manifested inside the system as user experience and when it fails it can have incredibly dire consequences. Legibility requires analysing and distilling systems and when those systems fail we have to rely solely on documentation.
And when that happens problems can happen.
So on January 13th in Hawaii, a federal employee accidentally sent out this message. And this was a false alarm.
There were no missiles en route, nothing bad had happened.
But let's look at how we got here.
So this is part of the software that this employee had to look at and go through. These are choices.
These are buttons.
This is the kind of software that the employee was picking from.
So Sid Harold who is actually speaking later in this conference and I'm extremely excited to see her talk, is a civic designer and former head of product at Code for America and she tweeted this in response, in response to the Hawaiian missile crisis: "The greatest trick the devil ever pulled was convincing the world that enterprise software complexity is properly addressed via training." (laughter) So legibility here is thinking that training would solve and overcome any kind of complexity, which I think most of us in this room know is not true. And we know this because there's a variety of examples especially the one I have specifically shown. But more importantly, if we go back to this, nothing in this UI is legible or understandable. Where is the logic, the hierarchy, the selection? Where was the confirmation UI that is supposed to pop up, prompting the user to reaffirm the choice that they're making? And in an interview I had with Harold she said, "I think it's true in the commercial world that interfaces for employees are rarely as good as the interfaces for customers." In particular when departments are strapped by resources and constraint and if you read stories about Hawaii for example, the government had three vendors you could choose from. And the requirements for that didn't include things like usability.
Usability and legibility are what make products and processes transparent and not adversarial. Which leads us to my next slide: when you think about impact.
So this is where auditibility now comes into the process. Auditibility is really actually about impact and impact needs to be meaningful.
Molly Soeder a researcher and author explained auditibility in an interview: "What we've lost in this rush towards transparency," she says, "is that we now have the ability to just see things.
But just seeing something doesn't really have an impact upon it." Auditibility builds upon legibility so users can understand enough to actually critique it. And think about the ways in which that system could be changed.
And for machine learning this is incredibly important. Removing bias from data sets and discussing data sets are often the suggestions for transparency.
But actually being able to audit a system and provide feedback that will be taken into consideration, having the ability to change may actually further push transparency.
And we see auditibility plenty of times in open source for example.
Auditibility can be filing a bug report or forking code.
It can be volunteering to create a process or product and have that product accepted and acknowledged in the ecosystem or service.
Auditibility can also be things like public forums where users or volunteers can voice concerns and see a response and impact from that.
Which brings us to thinking about how do we even put this into a process of data? And how do we think about the ways in which data can be impacted upon? So this is a process I've been thinking a lot about which is rating data sets.
And this is where I think we can think about even interaction or agency.
Because with interaction or agency you can take meaningful or rather, people can provide- people can meaningfully interact with a system, provide change to that solution.
And when they have the ability to actually impact what they're looking at, that's when impact becomes meaningful.
So again thinking about what does it mean to design transparency for machine learning? It's applying standards of legibility, auditibility, and interaction/agency to the data set being used.
Like how an algorithm is trained, what models the data is training on, and the product itself.
So a lot of what I've been thinking about is how do you bring this into a system? Perhaps it's providing different kinds of ratings. Data is what can determine the identity of an algorithm.
Bias learning products can come from biassed data sets.
Does a product itself set out to be biassed? Or was it using an unintentionally biassed data set when it was in train by one kind of person or one small team.
The data combined with how the algorithm is trained is what sort of creates these biases.
This is where information is key but including legible and understandable information. So what if all data sets came with a kind of rating? What if you could see the data set that an algorithm was trained on? What if you could have this kind of accountability? And this is what I advocate for when I think about or when I try to describe transparency with data.
And even here you can be transparently opaque. You're not necessarily describing everything inside the data set but you're describing enough of the data to where any kind of user can understand the impact of that data set and how it exists inside of a bigger system. Sorry, so I wrote a bunch of notes 'cause I wrote this talk a week ago.
This is something that's been bouncing around in my head a lot of what would it be like to actually try to put these into practise? And a lot of these different kinds of things I'm calling for can also sort of be shown as sub-categories of data or articulated in things like graphs and percentages and paragraphs. So there is this one demo I really love to poke at and I love to poke at it because it was designed by Google as a part of Google's jigsaw programme. It's called the Perspective API.
How many of you have heard of it? So Perspective set out with a very noble and important cause which was can you analyse toxicity in language? And as an online harassment researcher I'm sure that you know that I really really was interested in what this project could do or could not do.
And it's important to highlight here that inside the demo a lot of things are not shown. What you see is the likelihood of it to be perceived toxic but what you don't have is a breakdown about how the model was trained. Or what do the data sets look like? They do list the data sets they used.
They used four.
They used WikiData and the commenting data section from The New York Times, The Guardian, and one more and I'm forgetting which one.
But you don't get to see how old that data was. And commenting data can be very different from conversational data.
It's designed and it's in a certain space.
But I'd love to know more about how the system is rating this rating this language and more importantly how they are quantitatively measuring violence, how it's being paced and how it's being rated. Which brings us to this slide.
This slide is the most popular white supremacy phrase in the world. It's called the 14 words.
And in this case it's not perhaps so toxic. And again this is something that is more of a dog whistle.
It's not actually overtly racist.
It's not overtly toxic and it's not overtly violent. But when you know what it means, it's actually one of the most violent phrases in existence.
Which again brings me back to I'd love to know more about the data that Perspective was trained on. I'd love to see a bigger breakdown.
Where can I file my bug report? Where can I actually submit all the different research that I've done as a researcher at Harvard on toxic language inside of Neo-Nazi groups on Reddit for Chan? That's information that could perhaps be useful to this project.
But it's important as a user to understand when I'm interacting with a system, how the data or inputs or process by a system. And I, I really don't know.
And I can't audit this and I can't understand the legibility of it.
I mean you can go even deeper and I'm using perspective particularly here as a case study because I do provide some online documentation. So as you scroll down the page, this is what pops up and what's fascinating is that an engineer at Google really does maintain that perspective is an open source project and there is a fair amount of transparency around it.
But I would like to disagree.
I'm deeming it as proprietary transparent hybrid. And that's okay.
I think that that's fine for a lot of people in this room actually.
Not all of your code needs to be open source though it'd be great if it was.
But it's okay that things are not shown.
That being said when you're dealing with something that could be as charged as this example, or even extremely benign, and you're creating an API, well, how is it doing what it's doing? And that can be shown through a variety of different explainers.
So I've been following this project since early 2017. So as you scroll down and you look at this page, it tells you at the very bottom that you can go to the Get Hub page.
And when you go to Get Hub, what you see is this. So this is still the same demo but it actually changes names.
And this is where I think transparency starts to lose meaning in this example and becomes quite nebulous.
What does it mean to have something partially transparent but carry the flag of transparency? And this isn't a moralistic or ethical question. Not all code has to be licenced as open source. But rather this raises questions as to what constitutes as transparency.
So is this code legible? Perspective is the name of the demo and the accessible API but it's trained on something different. It's part of conversation AI.
Now this isn't clear on the about page and it's not super clear on this page either. So going further the about page is actually labelled for developers and in for developers, Perspective has a small line about being a form of conversation AI.
And then when you get to this page which is a separate page, there's more information about the project. But as we all know, if you send users to a completely separate page for new information you lose user traffic.
And the user interaction radically declines. So it takes two or three clicks actually inside of here to find the methodology that really explains how the conversation AI is trained on WikiData and it turns out it's majorly trained on this one data set.
It breaks down a very in-depth process that a seasoned researcher would understand. But is this really designed for engineers to plug and play with and to dig in? Or is it designed for something else? So what makes this transparent if it's designed for engineers who can understand high level research? Does that make it transparent? And I would say no because when you look at the demo it conveys a completely different experience. And this is important because when you think about the kinds of ways you're showing what sort of artificial intelligence system or ML system you're working on, what is the demo look like? What does you documentation look like? Those are forms of products.
I think design is an equalising action that can distil code and policy into understandable interfaces because design is the main way everyday users will interact with whatever you're building. This is where product design is explicitly and extremely important in the way in which you wrap your software into the world and the way in which it's presented to the world.
And I think, Sorry I lost my spot very briefly.
So how do we actually make things transparently? And as utilitarian as it sounds, I actually dream of dash boards in analytical UI and UX systems.
I dream of labels and warnings inside machine learning demos.
I wanna see visualisations of changes in the data corpus, network maps of words and system prompts for human help in defining yet unanalyzed words. What I imagine isn't really lean or material design. It's not minimal but it's extremely verbose. It's more of a cockpit of dials, buttons, and notifications.
Other than a slick slider that works only on mobile. Imagine warnings and prompts and daily, weekly, monthly analysis to see what is changing within the data.
Imagine an articulation a visualisation of what those changes mean to a system that is human readable. So imagine all of these kinds of labels that are given to an audience.
Imagine prompts.
Imagine how-to's and walk-thru's.
Imagine an emphasis on what is in the data set of the origins of the algorithm of knowing where it came from, of who created it, and perhaps why it was created.
Imagine the data ingredients that has a prompt for I on a label inside of your product that could be explorable for an everyday audience. What if all systems had ratings and clear stamps and large fonts that could say 'This is intended for use with humans and not intended to run autonomously, please check every few weeks.
Mistakes will be made.' Thanks you.
(applause) (upbeat music)