Artificial Intelligence: Making a Human Connection
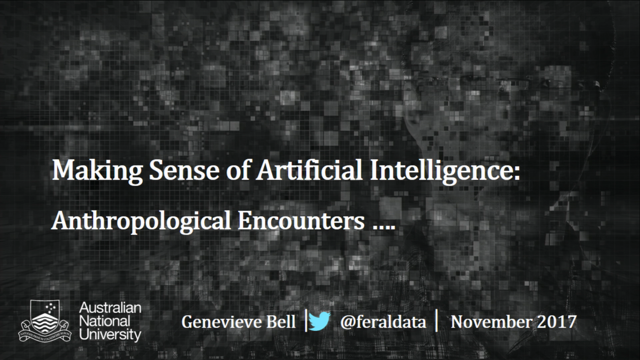
(upbeat music) - All right, I'm incredibly excited to get to be at Web Directions.
It's one of the conferences that I have the fondest memories of, and that event, in a very different building than this one, so many years ago, starting for me to think about what the kind of relationship was between the world that was coming of new technologies and where my discipline as an anthropologist sat. That's now four years ago, and the world has really changed in some significant ways, so I wanted to spend the next 50 minutes on the clock and counting trying to think about what an anthropologist would say about artificial intelligence and why that might be a worthy sort of form of endeavour. There are the usual caveats I should begin all talks with. I'm jet lagged.
I was in America four days ago.
That means I've had two flat whites, and for those of you who follow me on Twitter, you know I've had a jam donut, too.
That means I don't speak any slower than this, so keep up. All right.
So John gave you lots of ways of introducing me. I usually think there's a couple of things you need to know about me.
I am the child of an anthropologist, so I grew up on my mother's field sites in actually Indonesia and central and northern Australia in the 1970s and 1980s. I spent most of my childhood in aboriginal communities at a very different time and place, and in a moment where I mostly didn't go to school, and I went and hung around with aboriginal people who taught me all the things about this country that are remarkable, and it always makes it really special for me to come home and think about what it means to be back on country, so I kinda wanna pause here and acknowledge the traditional owners of this piece of the country and remind all of us in the room how lucky we are that we get to live in a country where we do that when we open at every single event.
Because that is not something they do in America. And that is always, for me, a kind of shocking omission. So I'm happy to be home on country.
I'm happy that I got to grow up on that country. So child of an anthropologist, it is a long way from (mumbles) in central Australia to Silicon Valley. I ran away from home, I ran off to America. I ended up at Stanford, as John said, getting my PhD in cultural anthropology.
My background in those days was Native American studies, feminist and queer theory.
You can see how a job in industry was an obvious next step in that career path.
And frankly, I didn't plan to end up in industry, I planned to be a professor, but I met a man in a bar, the way all good Australian stories go.
I met a man in a bar in 1998 and he asked me what I did, 'cause he was an American man and he didn't know any better, that that's not a good line in bars.
He said to me, "What do you do?" I said, "I'm an anthropologist." He said, "What's that?" I said, "I study people." He said, "Why?" (audience laughter) At this point, I should've (mumbles) the fact that he was an engineer, but you know, I went yeah, okay. (audience laughter) I said, "Because they're interesting," and he said, "What do you do with that?" I thought I'm really done with this conversation, but I'm relatively polite, so I said, "Well, you know, I'm a tenure track professor." And he went, "Couldn't you do more?" And I thought yes, I could stop talking to you. (audience laughter) And so I did.
And so it was unexpected when he called me at my house the next day.
'Cause we're talking 1998 and we're talking before Facebook, Twitter, LinkedIn, Tinder, Google.
There was no place where you could've typed redheaded Australian anthropologist and found me. I'm happy to report, if you now type that into Google, I'm the first or second search term.
So I have spent the last 20 years really doing good work for myself in the algorithmic logic space.
But no, he called me at home.
Now, my mother, who was a very sensible woman and gave me a lot of good advice in my life, told me not to give my phone number to strange men in bars. It's good advice and I had followed it.
So Bob got my number because he called every anthropology department in the Bay area looking for a redheaded Australian.
(audience laughter) And Stanford said, "Oh, you mean Genevieve, "would you like her home phone number?" (audience laughter) Yeah, 1998, we didn't know about data privacy in those days. So there was Bob on my phone saying, "You seem interesting," and me going, "You're not, please go away." And then, unfortunately for those of us in the room who haven't had an academic career, he then said the magic words that will always work for you, I have to tell you, 'cause he said, "I'll buy you lunch." (audience laughter) And I'm like, free food is always gonna do it for me, it is a sad thing to know.
So I had lunch with Bob, ultimately, I met the people at Intel, the people at Intel also seemed very odd. There's a much longer story there about why I would ever have said yes to them, but I found myself at Intel in the late 1990s.
I helped build their first ethnographic practises, their first UX practises.
I mainstreamed that competency inside Intel, and I spent the last five years there working in kind of, well, their strategic and foresight practise, I was their chief futurist, it was an interesting gig. And it meant that I've spent the last five years exquisitely paying attention to where the technology, the public policy, and humans are heading.
And it's that set of intersections that are really interesting to me.
And about where it is that the kind of propulsive force of the technology is heading and where it is, as humans, we're not always aligned to that, and how you might think about reconciling those disconnects. And it's in that context that Brian Schmidt, who is the vice-chancellor at the ANU, convinced me to come home.
He's rebuilding the ANU, he wanted to bring it back to its mission as the only national university in Australia with an idea of building national competency for the nation and of tackling Australia's acute and hard problems.
And he said to me, "I think we need to reimagine "what engineering should look like," and I said, "I think I'll do you one better, "I think we need to build something new." And so I came home.
For those of you who were raised in Australia around my vintage, you should imagine I'm currently regarding my life as one great monumental fang around the block. It's been 30 years, I left Canberra, I have come back to Canberra.
The planners tell me much has changed, I don't believe them. So with all of that as windup, what you should take from that is still operating on two coffees and a jam donut. And I'm really interested in thinking about the future, and in that particular context, for me, it's interesting to start thinking about AI. You can't pick up a newspaper in Australia at the moment or turn on the radio or the television without there being a conversation about this. Either in the context of coming job loss, in the context of autonomous vehicles, in the context of algorithms, good and bad. In the context of a data-driven world, there's been an enormous amount of conversation in this regard. I wanna suggest, however, that all of that conversation hasn't necessarily been setting us up to make sense of this as an object.
And in that regard, I wanna kinda pull it apart a little bit and think about, as an anthropologist, how would you approach AI? You know, and how should we all in this room, who think about this both as a technology, but also as a space in which we are going to express our work and also have our work expressed.
How might we wanna start to unpack and take this apart? There's lots of ways you can think about artificial intelligence and AI as a constellation of technologies.
It's clearly about things like machine learning, deep learning, algorithms, computer vision, natural language processing.
It's made possible by dramatic shifts in computational power and by the availability of larger and larger data sets. But it's also the case that artificial intelligence isn't just a set of technologies, it's also a constellation of social, human, and technical practises.
And as soon as you get to something that's that sort of ensemble, the human bit and the technical bit, that's my wheelhouse, and that's where anthropologists most like to play. However, as someone who grew up doing field work, I like to go hang out with people, and that's my kind of gig, right, I go spend time with people in the places they make meaning in their lives and try and work out what's going on there. It's a little hard to work out how you would do that with AI.
Well, I can go hang out with the people who were making AI, and that's an interesting, in some ways, ethnographic project I hope to get to embark on.
You could go hang out with people who were experiencing artificial intelligence like objects.
Apparently, that's all of you in this room attempting to hack ask the John bot.
You know, you are, in fact, having, at one level, an interventionist ethnographic encounter with AI, and I'll want to know about it later.
But that's not a scalable project, right? So I've been really struggling to think about how would you, as an anthropologist, start to unpack this. And so I went really old school.
I went back to a man named James Spradley.
He's an American anthropologist, he wrote this book back in 1979, after a long career of doing anthropological and ethnographic work.
In this book, he basically says that, in addition to participant observation, the deep hanging out that anthropologists do, one of our other critical methodologies is something that he calls the semi-structured interview. So think of this as being different than a questionnaire, different than a focus group, this is the notion of an interview, and what you're attempting to do is uncover how your research participant makes sense of their world.
Semi-structured because this isn't well-defined questions, this is not tell me your name, tell me your address, tell me what you did yesterday. I now wanna ask five questions about what you did with the PC and what you're doing with the ask John bot as we speak.
It's not that.
The semi-structured interview has a notion of there are categories of questions you should ask, not specific questions.
And those questions have intentionality to them. And Spradley says there are three kinds here. The first kind is what he calls descriptive questions, where what you want to do is get your research participant to describe their world in their own words. Because their language turns out to be one of the ways they frame how they make sense of things. So what are the choices of language they use to introduce themselves, to identify themselves, how do they describe who they are and where they're from? You all know how to do this, every time you introduce yourself to someone new, this is the bit where you say here's my name, here's how I got here. Here's how many coffees I've had, here's my Twitter handle. You know, we know how to do that, right, we have a set of practises by which we describe ourselves. So he says you have a whole set of questions where that's what you're trying to do.
Get to how people make sense of themselves. He says the second set of questions are structural questions.
And here, what you're trying to get at is how do people make sense of the ways they organise their world.
So if you were doing an anthropology of work, this might be where you ask how do you get to the office, what do you do when you get there, what tools and technologies are you using to get your job done? How do you describe your job, tell me what the last hard thing you did was in your job? If you're asking someone about their entertainment or leisure practises, this would be similarly walk me through how you find new television. How you shop for something.
And give me a framework not for how you describe things, but for how you start to make sense of them at a slightly more abstracted level.
And then he says there's a third set of questions, and these are, in some ways, the hardest ones to work out how to ask effectively.
Because what these questions do is ask your research participant to explain why they aren't like other people.
So we know one of the ways that human beings most like to identify themselves is why we're not like someone else.
Natasha, are you in this room somewhere? Oh, where's my favourite kiwi? I know you are, anyway.
Somewhere in this room, Natasha is here, she's from New Zealand.
Those of us who are in the room from Australia know full well how to sketch out the difference between New Zealand and Australia.
Apparently, at the moment, that means that, you know, we should be giving them Barnaby Joyce as some sort of present.
(audience laughter) And we know how to talk about the difference between Australia and New Zealand, although they are remarkably close culturally and remarkably close in terms of histories and many other things, we can make sharp distinctions between us and them.
And that's one of the ways we identify ourselves. Same way you would say, if you were from Melbourne, you were certainly not from Sydney.
I'm from Canberra and it's just pathetic, there is no contrast to that, it's just sad. (audience laughter) And we all know why we laugh at that joke, right? So three kinds of questions.
No specific question you should ask, but the work those questions should do are those things. So what would it be like to ask descriptive, structural, and contrast questions of artificial intelligence? It's a little tricky 'cause it's not like an AI thing you can run around and ask, and then we could ask John, but let's not.
We're gonna pretend we've asked him.
One of the very first descriptive questions is basically who are you? So what if we were to ask artificial intelligence about its name? And let's pause here and think about the name. And I mean this in the most kind of semantic, linguistic, semiotic sense.
Because look at those two words, artificial intelligence. How long ago must it have been where calling something artificial was good? If we were building and naming this now, we'd call it bespoke.
(audience laughter) Or organic.
Or slow, or local.
In this case, it's artificial.
What are the other notions that artificial is highly attendant to in this period of time, right? Well, what is artificial, it's in contrast to natural. It's, in some ways, a notion of things that are built, not just appeared.
It has ideas about structure in it.
Think about all the other things that were artificial in this moment in time, artificial sugar, artificial fabric, we're talking about NutraSweet and rayon and AI.
And you know, what's the contrasting point there, right? And then the word intelligence, similarly.
What is intelligence not about? Well, it's not about emotion.
It's not about illogic.
It is allied to words about reason and rationality. It's not about artificial human, it's about artificial intelligence, and it's a very particular derivative of that notion. And you see the same thing in a lot of the terms that play out in this space.
Machine learning.
Again, interesting, right, you take a human attribute and you stick a technology word in front of it, artificial intelligence, machine learning, computer vision. All of those are doing really interesting work in terms of how we immediately think about this object. So if you asked AI to name itself, it has to tell you a story about where its name came from. And by the way, that name has a history and a context. And it's a context in a very particular moment in time. AI is, in fact, that phrase is coined back in 1956 in the United States, where artificial was the best thing you could imagine in the immediate post-war period, when intelligence was a glorious notion and where this was deliberately a stake in the ground about a world you were building.
So knowing the name really helps, so that's the first descriptive question.
How'd you get your name and what is it? The second one is, of course, who raised you? Like, who are your parents? So artificial intelligence has a lot of daddies, that really shouldn't surprise you.
We tend to know one set of them in particular and another set not so well.
So as I mentioned, the term artificial intelligence is coined in the summer of 1956 at Dartmouth College in New England in the United States. It comes as the result of a 10 week conference that brought together a number of mathematicians and logicians in the United States with the explicit agenda of crafting a research plan around making machines simulate human behaviour. In particular, ideas about language, learning, and rationality.
The series of people who came together in that moment are mostly young, they have a silent godfather, a man named von Neumann, who is in the background of this conference, organising it.
They have a second participant in this conference who isn't actually there, but whose thinking pervades everything.
So if you were mathematicians and logicians in 1956 and you have set yourself the task of deciding you can build a technical system that will mirror humans' ability to think, you need something. What you need is someone who's theorised what makes humans think.
And in 1956, the group of people who were at Dartmouth went looking at one American behavioural psychologist for that answer, a man named B. F. Skinner. And you're all going who, here's the thing. B. F. Skinner is an American psychologist, he is famous from basically the 1940s onward, even a little bit before the 40s, he's someone who believed that human behaviours were a consequence of basically electrical impulses and operant conditioning. So your body was subjected to activity, electrical impulses went in your brain and something happened on the other side.
Effectively, he made the body into a machine. He rendered it mechanistically and instrumentalously. He's also someone who believed you could train humans to respond in certain kinds of ways.
Probably no surprise he spent most of World War II working with rats and pigeons, and he firmly believed that you could train them so you could train humans too, you just needed to change our conditioning. You could make us behave differently if you put us in a different set of circumstances, repeated those circumstances and rewarded us for different kinds of behaviour.
Now, if you're a bunch of mathematicians wanting to work out how to build humans, that is an excellent model.
It's just electrical impulses in, electrical impulses out. Now, of course, Skinner is himself a response to a whole lot of other work in psychology. Freud, Jung, Kinsey, things that were a lot messier. Things that were about the unconscious.
Things that were about notions of humans being more than just electrical impulses in and electrical impulses out, that we had an ego, a superego, an id, an unconscious.
That we were, in fact, not simply a machine, but a much more complicated system.
None of that is there in 1956.
What is also not there in 1956 is all of these people. So from 1946 to 1953 in the United States, a man named Norbert Wiener, who would go on to be a leading light at MIT in the 50s, convened a series of conferences in New York called the Macy's conferences of cybernetics. Now, delightfully, and I do not know what to make of this, that is their conference photo.
(audience laughter) So the next time you think about how to photograph an event, I say to you, cybernetics seance needs to make a comeback. 'Cause I really don't know, I mean, I genuinely don't know what to make of that, it really is the most extraordinary thing, and there are eight of them.
They did one at every conference over this seven year period.
Yeah, like, what were you thinking? And why did some of those very serious people agree to that photo? So what's interesting about cybernetics, unlike the artificial intelligence agenda of 56, in the 10 years before that, what everyone was worrying about wasn't about the artificial intelligence, but about the relationship between people and computational systems.
So what cybernetics was all about was the human-machine interface, and about what it would be like to be in a world where the machines had increasing complexity and increasing intelligence.
That turned out to be a really robust conversation, it also turned out to be slightly dangerous politically. 'Cause as that conversation unfolds, what becomes clear is that, in talking about human-machine interfaces, you start to be able to talk about social engineering and about transforming the way certain kinds of processes might unfold. That conversation in the 1950s in America started to feel a little bit too close to socialism. And gets shut down.
What appears in its stead is artificial intelligence. So knowing you raised you is, in this case, not just a descriptive question, but actually a question about history and politics and cultural process. And as John points out to me, the other thing that's going on here is one about geopolitics and about a different kind of cultural process. Because frankly, at one level, the person who really raised artificial intelligence is probably not von Neumann, who was in that picture there, but actually Alan Turing.
So Turing, who was sitting on the other side of the ocean in Britain, is still alive at this moment in time, has authored a series of really, in some ways, instrumentalist and provocative papers about machine learning and intelligent machinery. He has imagined that it would be possible for machines to think.
He has argued quite persuasively that the people who'll be most troubled by machines thinking are the smartest people, because they will have the most to lose.
And he has gone on to theorise what it would be like to think about thinking machinery.
Of course, as many of us know, Turing was also in a period in his life that was particularly complicated in the early 1950s. He was charged and found to be homosexual in a time that was illegal, he was chemically castrated as a punishment and he later killed himself. His work, as a result, in some ways, falls out of circulation for a protracted period of time. So you can talk about artificial intelligence through its many winters from 56 onward without talking about Turing.
So there's another piece that says the question of who raised you was never just as simple as who was your listed investor.
It's also who got written out of the record, what stories don't we tell, why is it that we can talk about artificial intelligence and forget to talk about cybernetics and intelligent machinery? Are all conversations about funding, funding agencies and politics? So even the descriptive questions aren't quite that simple. And then, if you go to, in some ways, slightly more structural questions and you say, well, who raised you? What raised you up, what's your history, what's your broader, in some ways, structural context? Well, the thing here is that artificial intelligence might've been coined in 1956 and the cybernetics agenda might've existed for 10 years before that.
But we have been talking about bringing machinery to life for at least 200 years.
In fact, 200 years next year represents the anniversary of the publication of Mary Shelley's novel Frankenstein, in which electricity brings to life Frankenstein's monster. We have been thinking about the consequences of new technologies creating and animating things not just in fiction, and Frankenstein is preceded by Gollum, is preceded by a whole series of other stories. We've also actually been thinking about it technically for a really long time too.
This is by far and away my favourite precursor to robots. This is an automata from 1736 by a man named Jacques de Vaucanson.
In my very bad French, it is called le Canard Digerateur, or the Digesting Duck.
This is a marvel of machinery in 1736.
It's a duck, I mean, let's be clear, so it's about this big. It could waddle foot over foot, so 400 moving pieces, it walked like a duck.
Its beak went (claps hands).
So you know, duck-like so far.
It made a slight noise.
Vaucanson, who was a man of great inventiveness, worked out how to use vulcanised rubber to create a digestive tract for this duck.
And the (speaks French language), you could feed this duck. (claps hands) The duck would waddle and then, wait for it, the duck did the other thing that ducks do. It shat.
This is effectively a digesting and shitting duck. Wonderfully, because Vaucanson could not work out how to fake digestion in 1736, it had precast shit in its arse.
(audience laughter) I'm fairly convinced that's why you have graduate students, or in this case, apprentices, there was someone's job to go get the duck poo and stick it in the duck's bum so that the duck could wander across the stages of Europe and entrance everyone, which it did.
Voltaire said of this duck, "Without this duck, "there was no glory for France." (audience laughter) That'd be a contrast thing right there.
So when we're talking about artificial intelligence, we can talk about the 56 conference and the cybernetic conferences and advances in computers and ENIAC and that whole history. But we also need to know that artificial intelligence has an intellectual and a cultural genealogy too. It has a history.
For as long as we could work out how to make mechanical things, we've been trying to make them look more and more like they were real. And our literaturicians, our written science fiction, our visual science fiction has been coded through with stories about what happens when things come to life. And most of it isn't good, let's be clear.
Kill John Connor pretty much all the time, come with me if you wanna live.
I've seen things you people cannot believe, I've seen attack ships on fire off the shoulder of Orion, I've seen C-gates glisten in Tannhauser Gate, et cetera. And it all ends in tears.
You know, we know the stories of what happens when things come to life, it goes badly.
We only have Asimov's three laws of the robot to suggest anything different, and I'd want to suggest that's science fiction, not science fact.
So part of our anxiety about AI is written into it from the moment it came into existence.
It has always had attendant to it a set of stories about what might go wrong. And so when you ask where you come from, as a question, it's not just did you come from a lab in Palo Alto, it's where did the idea come from and what else is attached and bolted on to that idea. And then you get to the other set of structural questions, which is tell me about your day.
What would it be like at this point to ask AI about its day? Well, every single one of you on your way here have already encountered it.
Whether you picked up your phone first thing this morning and logged into, I don't know, Facebook.
Your feed is driven by algorithms.
If you came here, and I hope you didn't, using Uber. If you came here using Uber, you encountered AI, if you came here on the train, you used AI, if you came here and you used a map to look at traffic, you used AI.
If you had a banking app, you've used it, if you are currently posting things to the Internet that is, in some ways, backended by algorithms which are the building blocks of artificial intelligence. It's already everywhere.
It isn't fully fledged, working on its own autonomous machinery, but the notion of algorithms that are automating processes is well entrenched in your existence.
Almost every app that sits in your phone is backended by some piece of it.
Whether it's about our dating apps, our mapping apps, our shopping apps, our banking apps, our news feed, our social feeds.
Those all have algorithms sitting inside of them, data being collected and things being automated. Now, of course, there are some interesting challenges to that, right, which is that algorithms are built on data. The thing about data, it's always in the past, it's retrospective, by its very nature, data is the thing that happened back there. Which means every algorithm is being trained on what already happened.
Now, there's a couple of interesting problems with that. Number one, not everything ends up as data. Two, most datasets are incomplete and only as good as the question you asked them to generate them. And they aren't always the way that we want the world to be. So you can train a machine on data to get to an automated set of processes, that doesn't mean you get to a world you wanna live in. This photo here is from one of my favourite shearing sheds in south Australia.
If you're from regional or rural Australia, you'll know to recognise that as the wall of a shearing shed. If you're from that part of the world, you'd recognise the names of the families that have been there for 100 years.
If you were from that region, you'd also recognise when the war happened because the names fade out. You'd know when the drought happened in the 70s 'cause there's less names on the wall.
You'd recognise the moment when we went from hand painting names to stencils.
You can actually read an entire history of the wall ecosystem in that thing.
If you plugged it into the current versions of Google photo recognition, it thinks it's graffiti. And at one level, it is, but at another level, that's local knowledge that comes from a very particular place and a very particular set of data. So as we think about what you do in a day for AI, one of the challenges is where did the data come from, whose world is it, what's being built and what's being left out? And how do we think about all of that as part and parcel of what AI does now and what it might do in the future? And what would it mean to start imagining some of the not so distant technical futures of algorithmic living that moves from the things that are in your phone to the things that would be in a building like this to the transportation systems that inhabit Sydney to notions of data-driven worlds that we aren't yet ready to think about or, in fact, regulate for? So if those are the kinda descriptive and structural questions, there is one question left, which is the contrast question.
And it's very hard to imagine how do you ask AI to talk about the thing it isn't, right? 'Cause at one level, the thing it isn't is us. But that's not the most productive question I think you can ask here.
I think a much more interesting question to ask about AI would be to ask the question of what do you dream. Because it puts an extremely pointed finger on the notion of AI, of could an artificial intelligence dream. And if it did, what would it look like? Will artificial intelligence create or just automate? And if it created, what would that creation look like? Well, so this is a piece of art by an artist named, and this is the one place I have to look at my hand, HYde JII.
He's Korean, South Korean.
He made this by hacking a Roomba.
And he took the dirt sucking vents and turned them into paint blowing vents and created canvases using basically the algorithm of dirt in your home.
Now, is that art? I'm not quite sure, it's something.
And it starts to point to a kind of, in some ways, uncomfortable notion of what will it be when algorithms start to produce new things in the world. Not just new forms of knowledge, but new ways of being and thinking and open up conversations.
What would it be to imagine a set of algorithms that weren't recommendation engines based on all the things we had done, but interventions? What would an algorithm look like that was about serendipity and wonder and discomfort, not similarity and preexisting activity? And what would it mean to ask the questions of AI of what aren't you? What is AI not? And how do we think about that, and how do you push on that very nature of the things it isn't as a way of thinking about the things that it is? Sitting inside feminist theory, there's a long-standing argument about marked and unmarked categories.
The notion that certain words have inherent, in them, assumptions about the way the world works, those are unmarked categories.
They're mostly revealed when you mark them. Best example I can think of, astronaut.
We say astronaut and then we say female astronaut. And we have to say female astronaut because we assume astronauts are blokes.
It's like we have to keep talking about the women's test in the cricket, because test team just assumes not women.
Marked and unmarked.
AI has a whole lot of unmarkedness about it, right? And how you explicate that becomes an interesting challenge. What does it mean to think about what's lurking inside AI that we don't know how to articulate that is the moral equivalent of male cricketers, or possibly not? But think about what are the words you have to put in front of AI to get at this contrast question? Australian AI? What would that look like? We know the first fleet of autonomous vehicles that have been tested here by Volvo have a problem with our kangaroos.
Turns out they modelled roadside danger on caribou. Caribou are an excellent proxy for cows, dogs, deer, elk, moose, sometimes wombats.
They are not so good for kangaroos 'cause kangaroos do this on two legs, caribou do this on four legs. Turns out this particular model of car just runs into kangaroos.
So not so much Australian AI.
But as soon as you put that word in front of it, you have to start saying, does AI have a country? And what is that country? And what would that mean to imagine that AI is not, as we all know 'cause how could it be, it's not a neutral technology, it's not a technology absent value, it's not a technology absent an imagination of where it functions best.
In that particular case of autonomous vehicles, the answer is not Australia, but somewhere with caribou. What else is gonna be lurking inside these objects right of what they are and what they aren't that we ought to be paying attention to? So where does all of that leave you? Well, at least from a kind of an ethnographic interview, I think it leaves you saying we ought to do a better job in asking about the technologies that are in our world and asking where they come from and pushing on their logics. And thinking about how we name things.
Names carry tremendous power, they also limit our imaginations exquisitely.
Every time someone says to you autonomous vehicle or substitutes self-driving car, there is a really interesting slippage happening there between autonomous, which suggests an object operating autonomously, and a self-driving car, which now suggests a self.
And that's an interesting linguistics slip, right? So how we think about names is hugely important. How we think about who invented things, under what circumstances and in response to what pressure and funded by who and in whose interests sound exhausting, but all turn out to be really important questions and things we ought to ask as developers, as human beings, as citizens, those are questions we should be critically interrogating. The stuff in our worlds.
And knowing what work the technology is doing, how that work unfolds, and what work isn't being done are, for me, all part and parcel of how we might want to be, in some ways, better builders of new technologies by understanding they're not new in some ways and by being clear about where they came from. And because frankly, in some of these places, these technologies are desperately in need of a critical theoretical intervention.
Which I know on a Friday morning sounds a bit much, but I think there are arguments here to say what would it be to ask questions about what is the world that AI is building? I can say algorithms backend your dating world, and you can all go yup.
And then what if I said to you they are normalising your desires? And what if I said to you some of the catalogue of technologies that sit on your phone are normalising the way your world looks, the way your world sounds, the possibilities that you're being exposed to? That in fact, what it is doing is creating a vision of the world most of us wouldn't actually choose to embrace if we wandered into a room and were given two choices.
So there's a piece here that says how do we remember, in all the seductive language that surrounds these technologies, that we also ought to ask more questions? Doesn't mean we shouldn't build them, it doesn't mean we shouldn't use them, doesn't mean we shouldn't work out how to do remarkable things with them.
But I think it does mean we should be asking different questions.
And so what does that look like, right? What would it mean to ask different questions and to give a different context? I'm giving you one way in, which is to use, you know, a fairly boring 1979 ethnographic template. There's another way too, which is to say how do we think about this in a historic context on a broader sort of landscape and canvas? The World Economic Forum published this chart about two years ago.
The Cyber Physical Systems on the end there you should think about as basically the AI world that we've been talking about.
When this chart came out, it was seen as being a useful kind of way of framing the world we're in now. As someone who spends her time thinking about people, I find this chart really troubling.
Because it says the last 250 years were entirely about technologies and there's not a human in sight.
It also tidies up all the work of that 250 year period into four easy moments that were driven by particular technological regimes.
It suggests that the one we're in now has this lovely history, so it's all gonna be fine. Yes yes yes, job loss, but shit, we've encountered it three times before we got through it and it was all okay. This gives everything this kind of really comforting, stabilising, normalising, happy framework.
And it comes from the World Economic Forum, so how can we argue? And of course, the reality is, looking underneath that tidying up is all that same complexity I've spent the last 30 minutes banging on about. It's all about complexity that says, well, okay, what was really happening in that first wave of industrialization? You can say it's about mechanisation, but what did humans do in response to that? What did that look like, what were the consequences of that, what was really happening there? And at one level, of course, what was really happening there was that we went from talking about steam engines to talking about trains to talking about railways to talking about transportation systems.
And as we scaled up and abstracted from the original technologies to these broader systems, it turned out, as human beings, we didn't entirely know what to do.
And we had to build new ways of being and new ways of thinking.
And we know what happened in that first wave of industrialization, that's how we ended up with engineers. And I can bag on about engineers all I like, but let's remember, the very first school of engineering on this planet was in Paris in 1794, it was the Ecole Polytechnique, and it opened less than six months after the King of France had been killed.
In that moment in time, engineering was a radical intervention.
It was a moment in time when the French emerging democratic regime said uh oh, we don't have a king, we kinda don't have any royalty left. We kind of pushed the priests to one side, we actually don't have an authorial voice anymore to structure our world, but we could structure it with science. We could structure it with knowledge, that knowledge feels immutable, it doesn't feel corrupted like the aristocracy.
What would it be to make that science our structure, the underpinning of our nation? And they built a school of engineering to make engineers, because engineers were gonna be the people who stabilised democracy.
So you can hear me be mean about engineering occasionally, but I think it's really important to remember, at its root, it is a fundamentally radical proposition. And one that blended science, as well as philosophy, ethics, law, and delightfully, English literature. Yay for the humanities.
As you go through each one of these waves of technological transformation, there were structural institutional responses. Whether they were from governments, universities, public and private enterprise.
So that second wave of industrialization, really, it's not just about the production line.
It's about the production of capital at a scale that had never existed before.
And the structural response, in some ways, is the invention of the first business school at the University of Pennsylvania in 1881, when an industrialist in Philadelphia came to the president of that university and said, "I'll give you $100,000 "if you make me a better bookkeeper." President of the University of Pennsylvania was not a foolish man, he went, "I will take your $100,000, but I don't think "you need a bookkeeper.
"I think you need a new way of thinking about things." And the Wharton Business School was born out of that moment. And we can have our scepticism about MBAs and business school education, but that business school created, for better or worse, the GDP and the whole notion of measuring economic success that way.
They created the first notions and theories about how you manage labour and workers and the delta between workers and management. They created the first branding schools and the first branding experiments and the first market research.
So coming out of that moment of technology created a whole new way of thinking about the world, 'cause that technology produced, in some ways, a whole new system that needed to be regulated. Same is true when computing breaks.
So the first computers in the world are in the 1940s, they're really just electronic calculators. By the time we get to the 1960s, those have become increasingly sophisticated machinery. They require programmes, most of those programmes are proprietal.
So there is FORTRAN at IBM, there is FLOW-MATIC and COBOL at Rand and GE.
And the American government had a moment of going huh, how do we feel about the fact that the machinery we are buying, because at that point, the United States government was the largest single purchaser of compute power on the planet, how do we feel about the fact that we are buying things and we only can use the programmes that come with them? Is this a critical path failure, and if it is, what do we do about it? And so there was an approach made to a mathematician at Stanford University.
And the request was, okay, we know you guys are experimenting with how to think about computers at an abstract level.
So not just about these individual machines and these individual programmes, what would it be like to codify that? Could you make a curriculum to train people who could be in our camp, not in IBM's, like what would it be to do that? And the mathematician they approached built the first computer science curriculum. His name was George Forsythe, he finished that project in December 1968.
And he launched it at the Association for Computer Machinery in San Francisco.
He turned up with a curriculum.
And 30 other people that had worked on it with him from around the United States.
And there were 1,000 people at this event.
And he basically turns up at a room remarkably like this, puts down the curriculum and says, "Here's computer science." And everyone goes, "Thanks, that's great." And they take it back to their home universities and they start computer science departments. And every two years after that, the ACM updates that curriculum, and everyone goes, "That's great, thanks," and on they go.
Now, I don't know about you, but I'm an anthropologist. And I don't know of an anthropology department on this planet who could agree internally about what the curriculum should be, let alone agree on a planetary scale about what we were gonna teach as anthropology.
So the fact that computer scientists do this is, as far as I'm concerned, this remarkable structural act that one should be deeply admirous of.
Because it's just, it boggles my mind.
Anyway.
So that's the response, right, driven by a sense that the technology was creating, in some ways, a structural challenge.
And what was the institutional response? So you can give AI a backstory, but what's its forward story look like? What is the infrastructural and structural response we should have there, right? And what is the thing that is being created that will ultimately require managing? If the last ones were about steam engines and capital and computation, what's the next thing? I think it's data, pretty obviously, that is the mana on which all algorithms are built. It is the thing that will need to be managed. But the challenges here, much like the challenge of steam engines, it's not about managing the steam engines anymore or the data, it is about the technical system that is emergent on top of it.
So much the same way we had that steam engines, trains, railway moment, we have a set of technologies that are not yet a system, but are getting awfully close, of which AI is one, but so is IoT.
So arguably are some of the component pieces of AI, big data, algorithms, machine learning, deep learning. Those things at the moment feel like individual pieces. But it would be my argument, I think, that they are rapidly becoming a system and that that system will be characterised by scale and abstraction. And imagining what the institutional response will be is tricky.
Trains really shouldn't be managed by people who were good at thinking about steel under pressure. We had to create engineers to do that work. Bookkeepers were great, but you didn't want them thinking about how you manage stock options and companies. Electrical engineers, some of my favourite people, are really good, but you don't want them thinking about computers, at least not programming them. What if we're at that same moment now, apology to all the computer scientists in the room, you are my people. But what if you are not the people that we need moving forward, or not the only people we need moving forward? What if we're at the next moment where we need to think what is the institutional response to an emerging technical system that will be characterised by scale and abstraction? And that's how the vice-chancellor of the ANU convinced me to come home, was he said, "That's right," that argument you've just rehearsed with me. "And what are you gonna do about it?" I said oh shit.
The other piece of advice my mother gave me. Never tell people you're gonna do something, 'cause the worst thing that will happen is they go excellent, get on with it then.
So I said to Brian, "I think what we need to do "is build a new applied science." I think the moment is here to say we need to build the next thing, the next engineering, the next business school, the next computer science.
We need to build the apparatus and the intellectual set of questions to tackle that world. Here are my caveats at the very end of this 50 minutes. I don't know what it's called yet.
We didn't know engineering was called engineering until 50 years later.
Computer science was a little quicker.
I don't know what it's called.
What I do know is that it turns on three questions or three vectors that I think require interdisciplinary exploration and that will ultimately adhere to a new body of knowledge. And those three questions are formulated around three what appear to be technical ideas, but in fact, when you push on them, are much more complicated. The first vector is around ideas about autonomy. What do we mean when we talk about autonomy? At the moment, we talk about self-driving vehicles, that's one vector in autonomy, but it's clearly already bringing to bear an entire body of philosophical knowledge that is probably worthy of scrutiny.
The notion of autonomy and autonomousness and its links to sentience and consciousness are ideas that Western philosophers have spent 2,000 years arguing over.
And it is well rehearsed terrain.
It's also the case that autonomy is, in fact, a loaded cultural term.
There are other cultural systems where autonomy is not a thing, it, in fact, doesn't exist.
If you look at some systems, it's actually about co-emergence, it's not about the notion that there are single individual actors, there are co-emergent systems.
What would it be to say maybe the characteristics of a data-driven world are not about things being autonomous but, in fact, about something else? So how do we move through that idea and open up space for both new technical explorations, but frankly, the notion of granting a technical object some degree of autonomousness has huge implications. Public policy, the law, ideas about security and safety. Even how we think about that as human beings is a little bit complicated.
And it goes back to the history of technologies that come to life, it goes back to the early history of robots. Even the word robot itself has written into it a notion about the relationship.
The word robot is an invented word made up by a Czechoslovakian playwright in 1920. It is a derivative of a Czech word, the Czech word is robota, which means serf or slave.
Yeah, exactly, so sitting inside the technical object is already an idea about its relationship to us. And as human beings, we don't have a particularly good track record of thinking about things as being autonomous, even inside the human race.
We have certainly, in the arc of the history of most of us in this room, lived in a world where women were less autonomous than men, where children are granted limited autonomy, where whole other categories of human beings are not seen as having autonomy.
Where notions of relationships between us and things we grant autonomy is writhen through with ideas about inequity, politics, notions of otherness.
And while those sound like ideas that aren't in our technology, go look at it and you'll find that they're all there. So how do we unpack and unpick that idea and think about what the consequences are? Second vector, I think, is hugely important in this data-driven coming world, is one around what it means to say, if the object is autonomous, what are the limits on that autonomy? How much agency does it have to go do things? We grant that people under the age of 18 are autonomous, there are a whole lot of things they aren't allowed to do. Vote, drink, vote, you know, we have limits, right? What are those limits gonna be on these technical systems, how do we think about it, how would we manage it? And what happens when it's no longer a one to one scale? At the moment, you may have objects that are acting on your behalf.
What happens when they're acting at an institutional scale? What happens when it's the web directions bot negotiating with the Carriageworks building about the price of coffee and the absence of donuts. At what point is John gonna go, you know, that's just not good enough? And at what point does John get to have visibility into the algorithm that is working on his behalf and know how it was trained and what its decision making is? So where's the transparency and how do we even think about that? And then last but by no means least, if you imagine that you can solve the problem about what does it mean to have a technical object that is autonomous, a technical object whose parameters you have determined, how do we think about assurance in that space? How do we think about safety, liability, risk, trust, privacy? How do we think about what a world will look like when there are technical objects engaged with each other and not with us? What will it mean to feel human in that system, how will we think about the limits of safety, how will we do that knowing that some of these technical objects aren't built here, knowing that there are different standards on the globe for everything, and this is not going to be the place where we suddenly get to a global standard. I hate to tell you that.
Like we haven't solved it for electricity, currency, or anything else, this is not going to be the sudden place where there is miraculously a world standard for artificial intelligence and we are all happy and it is done and dusted. What you will, in fact, have is multiple different state regimes and state notions. And how we think about what we want those to be here and in every other place we come from is actually an open conversation right now, and thinking about where we want that locust to be is hugely important.
In my very kind of overly ambitious self, I've put up my hand to go solve these problems, I've given myself five years.
I have two to get the lit review and sense making project done, and I have three on top of that to get a curriculum out to the planet.
That means a couple of things.
I'm busy, I'm hiring, and I need help.
Because the thing I know is you can't do this by yourself, and frankly, I don't want to.
So if you go away and think, "That woman is crazy, "but I'd like to play," I'm really easy to find. You can find me on Twitter, you can find me on email, it's just genevieve.bell@anu.edu.au.
You could still find me at Intel, but please don't. You know, or you can just turn up in my office in Canberra, that would be good too.
So with that, I want to, two last things.
One is say thank you.
The second is, on behalf of my producers at the ABC, I need to tell you you can find the Boyer Lectures wherever you get your podcasts or on the ABC Listen app or at abc.net.au/boyerlectures.
And I think that's everything, so thank you. (applause) (upbeat music)