Bringing Design, Product and Data Scientist together to design intelligent product
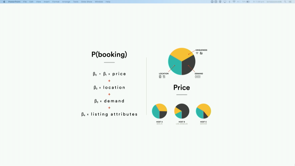
(upbeat pleasant music) - [Larissa] Okay, so before I start, how many of you are currently working as data scientists? Quite a few, what about behavioural scientists? Less so.
Okay so today...
just get set up.
For the last two years my teams and I, have been working with data scientists and behaviour scientists to help customers to bridge that gap between what they intend to do and what they actually do, and by doing so helping them to get themselves in a better financial position as well create better homes for themselves. That collaboration between designers and data scientists and behaviour scientists is really changing the way we work and it is enabling us to have a bigger impact on customer's life and I'm going to give some examples of that. So today I'm going to talk a little bit about design and data science, a little bit about design and behavioural science and then I'll share my experience in bringing those new disciplines together. So, a little bit about data science.
I'm gonna use this.
So the so-called general AI, this machine that can take information from different domains, can engage reasoning and understand how we feel, this is still years and years away.
However, we have made great progress in machine learning which is a subset of AI.
They use models and data to perform tasks without being specifically coded to do so and that is a very exciting time for us designers. However, most of us in this room work with data scientists, are trying to achieve very simple tasks in one domain and it feels more like that.
If I can play it (chuckle) It's thinking.
Oh good, there you go, He explains how it is, very, very clumsy (laughs) Even when...
(laughter) So, it is very exciting because the reality is machine learning is enabling us to do personalization in a much, much better ways than we could before.
So we have better recommendations, better search results, better notifications and I'll give an example of that shortly.
We have now new ways (clears throat) excuse me, to interact with customers because machine can understand what we say and can see, what we see.
So we can ask Siri to you know, set a reminder, we can ask Google Photos to create an album about my cats.
And there are other things that machine learning is enabling with vision as well.
I have a friend who is blind and she bought herself one of those glasses that has a little camera, it's called OrCam, and what it does, it reads for her from a physical paper, it also tell her when someone, they're familiar to her is approaching so it's life changing stuff.
Machine learning is also very good to provide insights. So we can look at behaviour data and group people of users and offer experience of features specifically to those users which can be quite handy.
I've worked with a data scientist using, analysing the traits of our personas and then using them to identify and size them into our user base, using behavioural data, which was pretty cool because you actually could confirm those personas and size them.
So it's quite powerful from a design point of view. And the last one that's really relevant to us is predictions. It's the ability of preempt what people will do next and being able to serve their experience before they ask it. At least I guess, giving them less options so it's not everything. Today we can predict what each one of you is going to spend at Christmas, and if you did that in advance we can help you to save up for it. So it's quite, powerful what we can do with data these days. I'll give some examples, this is Will Ferrell and Chad Smith, Will Ferrell is a famous actor, Chad Smith is the drummer of Red Hot Chilli Peppers and they are incredibly alike.
Machine learning can identify them with an accuracy of 85%, which is pretty amazing.
If you see a photo of them, it's really hard, it's like twins.
Another example that you'll be very familiar is the Google prediction text.
It's getting really good, really fast.
Can you imagine, five years from now, like we will wake up in the morning, all the emails will be ready for you to send, right? So it will be quite cool, I'm sure there'll be other problems (laughter) with them, you know.
You can imagine the impact of them as time goes by. Another favourite, Google Translate dynamically translates over 20 000 languages, and if you couple that with Computer Vision, so you have the experience that some of us, or a lot of us would have done overseas.
I was in a trip overseas with my family recently, and so I was sitting in a little cafe, and we ask for a menu in English because they didn't have the menu in English and the guy took his iPhone from his pocket and give us Google Translator which I thought was quite funny.
But, it makes sense, why do translating in different languages, especially if you are in a popular destination and we have visitors from everywhere? So we just used the Google Translator, and so it's funny that he's also using his own phone but maybe that's a cultural thing.
One of my favourite examples, is Spotify Discover Weekly, who knows of this feature? Lot of people, yeah you use that.
It is a recommendation engine and what makes this quite special is that it use three strategies to make the recommendation. The first one, is comparing, looking at your behaviour and others, and people like you, like Netflix, but unlike Netflix they use their star rating, it uses implicit behaviour.
So, how many times you listen to a song, have you saved it in a playlist? Have you looked at the Artist's page after listening to the song? So use that implicit feedback on things you like to build relationships.
The second strategy is analysing text, and so it combs the internet looking for articles, blogs, comments on artists and songs and use those text relationships to associate things that are alike.
And the final one which is, I guess the secret sauce is, they use the music track, the audio track, raw data to analyse likely.
And what is special about that is because it's the only analytics tools that don't use popularity.
So it's great to find new music that no one knows about yet so it's pretty clever.
And I do feel for, you know some of the other players and especially Pandora in the early days, the fruit of love but there's no way a human could do that, just no way. So very exciting times from a technology and, the other other discipline I feel like, you know, I have been lucky to work and get to know quite a few Behavioural Scientists, they're quite smart, amazing human beings.
Even if it don't work with them, I think Behavioural Scientists coming to our practise through different manners, there's behavioural design and gamification use a lot of that kind of philosophies and principles.
Behavioural sciences, they are cross disciplinary, so borrows from different science disciplines to understand how people behave, it's about understanding people.
And what Behavioural Scientists do is, using the techniques and the principle of Behavioural Science and Cognitive Science to introduce the concept of a more realistically behaviour of humans when you design products for them. And whenever possible, helping them to make better choices for themselves.
They are important to Behavioural Scientists, is their agent, to not remove their agency of the users, give them choice and options and let them choose but help them in that process. For those looking to introduce some concepts of Behavioural Science, Behavioural Science, this is a quite good framework, it's a long paper, this is very snapshot of it, it's called EAST, it's produced by the UK Behavioural Insights team. E stands for easy, A for attractive, S for Social, and T for timely.
So this is kind of the basic concepts of how human behave.
Easy is around, you know as humans we have limitations, we can only process so much.
We forget things, you overlook important information so what is really important, is that we harness the power of the thoughts, making, you know, simple choices.
We send reminders and we simplify methods, we also make things stand out, the important information to stand out.
The second one, being attractive, it's not only looking good, which is, we know from research looking attractive means that people trust more or think the usability is better, just straight off being human, obviously there is no correlation between those things, however, as humans we do that correlation.
But it is also about how we present information, how the environment and how we position things really influence decision, so we have to really think through how we frame things and, also how we sort of plan and design the environment of choice.
The third one is social, we are social beings, we look for social clues from people around us that help us to make decisions and you know, guide our behaviour especially in an environment that we are not very familiar with.
In that, kind of harness the power of, you know, social agreements, good things like, good examples of that could be Dry July, so when you make that commitment as a group. The Movember thing, November, Movemeber is another one sort of like when you do that public commitment, when you do as a group, it's more likely that you achieve it.
And the fourth one is timely.
Part of it is being able to identify the time that people have better propensity or to respond to your message, or to you offer.
So the same offer at different times can create totally different outcome so you have to experiment a little bit with that because time matter, timing matter. The other thing is about, is like as humans you are very very bad at projecting ourselves five years from now, 10 years from now, 15 years from now.
It's something we aren't very good at, projecting and making these trade-offs now for then.
So when you design and you have those trades-off, like in retirement or health issues, you need to bring the trade-off for day to day, like the little choices you make today rather than big choices that will have huge impact in the future.
So, little example.
This is a classic case-study from the 90's, it is the airport of Amsterdam and the Cleaning Manager has got very very frustrated with the state of the boy's toilet.
So he came up with the idea of putting the little fly there and the result is, 75% reduction of cleaning cost.
That is what I mean, just little change in the environment in the context can influence behaviour.
Sorry, this is another example, this is the US app from CBA.
The aim of the US app is to, increase financial literacy in young kids and we did that by teaching them the concepts of saving, spending and creating goals, and also sort of the trades-off. So the first behavioural influence that you do is, we did, was I encouraged them to create a goal. Again, that commitment vice, we know if you explicitly commit to something it's more likely that you achieve it.
So encourage them, they could go without a goal but, you know, encourage them to create a goal.
And every time they want to spend money, they actually need to move the money from the saving account into a pocket money or spending account, so trying to sort of separate this concept of saving and spending.
And every time they did that, that thing will come up, the burn chart of your goal, the time to get to your goal. We know from our trial that 30% of the kids that hit the page during transfer.
What we're teaching them is this life skill of delaying gratification, so you know, just by design, by design which is quite cool. This time this app is live and you can get for your kids if you have them.
Very similar disciplines, you know they are quite distinctive in itself but you know there is a lot of overlap between Data Science, Behavioural Science, user experience and product management. And we know that, if you get them together you'll be a lot stronger.
How did I go about getting it together? So my experience has been, actually before that I'll tell you a story. Collaboration in workplace, maybe it's just me, can be a war, it can be really hard especially when you bring people from different disciplines together. They have different ways of working, different ways of thinking and you know, it's really hard to kind of get them to work with others in a different context.
So that's the environment we actually had when we first started working with Data Scientists and Behavioural Scientists.
On one side, we had the designers iterating and creating experiments, like in a really fast pace and then came the Behavioural Science and they want to review all the literature. Everything that have ever been written about this subject needs to be read and researched and we make sure that we're not going over things that were approved and someone did good research on.
And in the end, you have to set up some experiments which is very scientific ways of doing things, so our experimentation were like trial and error, it didn't work.
And then you have the Data Scientists, they, broadly at that point, was working in different engine, sort of in a different group, in a different area.
So sort of, it was really hard to bring to the fold, really good people, everyone interesting, doing their work together but really hard to bring together. So what we did, we started this concept of joining the player journey, which is a gamification kind of concept where your first step, you onboard people.
You really just spent time understanding each others discipline, what do you do? How do you go about it? What does that mean, etc.? And after you do that, you get them in a project together to do some work together, in that process they start to figure out what each one brings to the table.
And then those processes that work can start creating new habits where they didn't exist and those habits become processes and before I know, you've got some teams working really well.
So a really nice way of getting there without being toads.
There are few things that also work for us. First one is building on the existing process. The design process is well established in the organisations that I worked for, especially the previous one.
So when we brought them in, we're kind of trying to bring them to our process, that didn't work so well because they didn't, kind of us imposing to them but we find ways over time, to get that working. So for example, when we started work with the Behavioural Scientists, there was just one or two of them so they had like genius bar where we would go and show designs to them and they would give us like amazing advice, really quite insightful suggestions.
But they also gave this feedback, look we can't really do much when you actually have already solved the problem, we need to work together a lot earlier.
When we're trying to define the problem and trying to define which behaviour we want to change, so we start to, over time, bring them.
That team grew so there could be more dedicated to project so we start working with them in the problem definition, which really changed the dynamic in how we worked. While the Data Scientists, at the beginning they didn't want you to go, it's like, there's this huge problem, you know, it's really hard to break, can you help us? They go like, I can't help you, like you have to tell me exactly the data we want, the problem we're trying to solve and I'll see if I can get those datas for you. Which is interesting because my most recent experience is that the Data Scientists are actually being quite proactive in looking for ways of solving business and customer problems by using data.
And then you can collaborate them at an earlier stage so I think that there as been a huge progress in that community, in the last two years.
Design Sprint, who has not used Design Sprint? No one, all of you have used it, good.
Great way actually to get that discovery thing, really worked for us.
Five days in a place, working together sort of hustling with a problem and getting an outcome at the end of it, and they say, oh we actually can work together. Actually, there is a way between us to collaborate, so a very good way, we used Design Sprint for different things but if you never work with the other disciplines, it's a good way to get it started.
Co-location, very hard because in general, Data Scientists and Behavioural Scientists are spread thin across multiple projects so it's really hard to have one-to-one in teams but when that happens, magic happens because you can move a lot faster than the standard problem.
You look at the data, it's a lot more experimental so I highly recommend, we have a couple of teams they have Data Scientists at Hipages they're embedded in to make a difference in the pace and the quality of the work as well, in the collaboration. Common language, (foreign language) okay, that's exactly how we started, we just did not understand each other, by the way this is Portuguese. I think it's necessary to spend some time trying to understand each discipline, in the same way we are very good at educating others about design, you need to be prepared to be educated about other disciplines.
And it's not too hard and Data Science is not too hard, the coding bit of it I think is pretty hard, (chuckles) but the concepts are not that hard. You just need to break into chunks and try to learn what the techniques they're using, how does that happen, what the algorithm is looking at. It's really important because then you can establish a conversation.
It's the same with Behavioural Science, can you pass this sort of, the EAST framework and start understanding behaviour in a deeper way, and some of those influences.
It really makes it a lot easier to work with others if you actually understand their disciplines. And I recommend, if you have Wiki's to document those things so it's available for others or Playbooks, if you use those.
Here's an example of of Airbnb, this is, if you are a host, they have a feature where it's called Smart Pricing.
So they use different, I guess variables, to recommend an ideal price for your property.
So if you look left, it's the algorithm of it, so it use price, location, demand and listing attribute.
So the prices, the location, demand and listing attribute. Listing attributes is how unique is your property. On the right-hand side is how business see it.
So if you look at the chart you see like it is in Los Angeles, they are seeing them, drives price is demand a lot of people go to LA, while in Denver what drives price is uniqueness of the place. So that is the language of the business, the language of the Data Scientists and for the users it's simply saying it's based on listings qualities, nearby prices and demand. It's really simple, so it's the same thing, they are talking about the same thing but they use a different language when talking to different. I love the example because it creates a lot of transparency of how the algorithms work, in fact, at Hipages we are developing a very similar concept with how our price work because they vary.
And it is should be in market very soon so I'll be able to share soon.
Okay.
Another thing that helped us, sorry, went too fast, wrong direction, back, back, back, back.
Collaborate on experienced principles.
Anyone that did experienced principles know how hard it is, this actually takes a lot of work to synthesise what it means, what it means to your own product, what it means to your company, broader principles of design, bringing all of things together.
It's quite involved, that requires a lot of collaboration, so it's a good exercise if you bring in those, discipline to your group, to spend time to them to understand how their discipline is going to influence your principles.
So in a couple of examples, one is design for humans. So talking some of the principles of Behavioural Science into account.
And the second one is humanise the data, so it's again, how we're going to treat the machine learning aspect of our machine, of our product.
Just sitting with them and working through workshops make a huge difference, so I highly recommend.
Collect data for the future, really important thing. Most companies today, collect as much data as they can, one because storage is cheap and two because they know that data can be commercialised and data is also power.
The problem with that, when you collect data in a non-structured way, is that it is harder to use it later.
So if you have a notion where you're going, if you have a vision, it's worthwhile to sit with your Data Scientist and Data Architect and actually plan for that.
So this is an example of something we are planning for the future, and some of the data we don't have now so we need to start to think how we're going to collect those datas, how we're going to structure it, where does it go, what's some of the implications. So we have a quite, that's very unsexy, I'm sorry but it's a very big spreadsheet just in each concept for their use to plan for future needs. And then final, I guess, advice is start small, it's not necessarily where we started. We started really complex and worked really hard and we really had to actually shape and we really had to get momentum so when you break things in smaller things, like that simple step, one thing, it's when you start to create a method of working that then you can scale later.
So I will leave you, (bubbling) It's just not the same without the sound.
(laughter) (bubbling) (laughter) (glass shaking) (explosion and laughter) Well it's not that bad but it's all about experimenting different things and get out there and just have a play with it and try different things because these disciplines are here to stay and we need to get our head around it, thank you. (applause) (upbeat pleasant music)